AI Technical Due Diligence plays a vital role in the development of AI-powered solutions. It involves comprehensively examining the system’s architecture, algorithms, models, and technologies employed. The main goal of the technical analysis is to uncover both the strengths and weaknesses of the AI solution and identify any potential threats and risks that could arise during its implementation.
In this article, we’ll explore a technical due diligence checklist, highlighting the key areas that require your attention. But before we dive in, let’s address why tech due diligence holds such significance.
Why is tech due diligence important?
Tech due diligence plays a crucial role in ensuring a solid foundation for your AI-based solution. Some of the key benefits include:
- Risk mitigation: AI Technical Due Diligence examines AI technologies, models, and systems to identify and address potential risks and challenges. It enables proactive mitigation strategies, minimizing costly failures or setbacks.
- Cost efficiency: Thorough AI Technical Due Diligence is essential to avoid costly mistakes in AI investments. It enables accurate budgeting, efficient resource allocation, and cost-saving opportunities and maximizes ROI.
- Informed decision-making: AI Technical Due Diligence offers insights into an AI system’s strengths, weaknesses, and limitations. It facilitates informed decision-making, ensuring the selection of suitable solutions, realistic expectations, and alignment with business goals.
- Competitive advantage: The process provides insights into your AI system’s capabilities relative to competitors. Identifying areas for improvement and leveraging strengths gives you a competitive advantage, allowing you to capitalize on AI technology opportunities.
- AI compliance: AI tech due diligence helps ensure compliance with applicable laws and regulations related to AI technology. To learn more about this particular topic, refer to our article on the legal challenges of AI.
- Investor assurance: Last but not least, technology due diligence instills confidence in investors, stakeholders, and partners by demonstrating that thorough analysis has been performed and potential pitfalls have been considered.
Key elements of AI Tech Due Diligence
Vital elements of AI Tech Due Diligence include validating AI concept feasibility, assessing technical standards, verifying training data quality, reviewing AI stack components, revising datasets and models, assessing legal compliance, and more. We delve into each element in the following section.
AI Concept Validation
If your startup is till in a concept stage, AI Concept Validation involves conducting preliminary assessments and experiments to determine whether the AI solution you’re planning to build is feasible, technically achievable, and aligns with your objectives. In addition, this process helps identify any potential challenges, risks, or limitations that may come up during the project.
The goal is to gather evidence that helps you make informed decisions about whether to proceed with further development, investment, and implementation of the AI concept.
Technical Validation
Technical Validation in AI Due Diligence involves thoroughly assessing the technology, algorithms, models, and infrastructure to ensure they meet the required standards and specifications. Your AI system is put through a series of tests, analyses, and examinations to validate its performance, accuracy, reliability, scalability, and security.
It may involve reviewing the code, testing the system’s functionality, evaluating its computational needs, examining how it handles data, and assessing how well it can be integrated with other systems.
The main goal of Technical Validation is to evaluate the technical feasibility and effectiveness of the AI system while identifying any potential limitations, vulnerabilities, or gaps that could impact its performance.
AI Training Validation
Once you determine the viability of your AI-based product, it becomes crucial to evaluate the training data’s quality, relevance, and representativeness. This step ensures the data accurately captures real-world scenarios the AI model will encounter. The process involves validating datasets or employing cross-validation techniques to guarantee that the AI model has been trained on a sufficient amount of reliable data, thereby minimizing the risk of inaccurate generalization.
The objective of AI Training Validation is to identify any potential issues or biases that could affect your system’s performance.
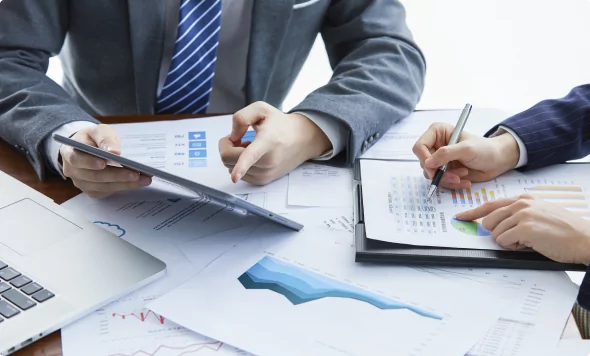
AI Stack Review
AI Stack Review comprehensively evaluates the technology stack used in your AI solution. It includes assessing various components like architecture, codebase, frameworks, libraries, tools, and infrastructure. During the Stack Review, AI consultants also look into data-related aspects such as storage, preprocessing, and machine learning algorithms. Additionally, the review may consider factors like licensing, dependencies on open-source software, documentation, and following industry best practices.
The purpose is to assess the AI stack components’ suitability, efficiency, and scalability and identify potential bottlenecks, performance issues, or security vulnerabilities. Furthermore, the process involves optimizing the models to ensure cost efficiency, which is particularly important when utilizing external hosting services.
Bias and Discrimination Verification
Above all, the AI systems must comply with privacy and data protection laws and be taught not to discriminate. The problems may arise when the data used to train the system is unrepresentative or contains inherent biases. These biases can manifest in various forms, such as favoring certain demographic groups, perpetuating stereotypes, or disproportionately impacting marginalized communities. Conversely, discrimination refers to the unfair or unequal treatment of individuals or groups based on race, gender, or age.
The aim of Bias and Discrimination Verification is to evaluate the impact of the AI system on different user groups and ensure it promotes fairness and inclusivity.
Dataset and Model Revision
In Dataset and Model Revision, you examine the datasets already used by AI algorithms. If your system has already been functioning for a while, it might need improvement or adjustment to the changing data sources and market or regulatory conditions. The revision involves examining the data’s quality, relevance, and representativeness, as well as evaluating the AI model’s performance, accuracy, and reliability.
The purpose of Dataset and Model Revision is to verify that the model is well-designed, adequately trained, and capable of producing reliable and trustworthy results.
Legal and Regulatory Compliance Assessment
As we mentioned in our previous article on AI legal challenges, regulatory issues shouldn’t be taken lightly. Although AI development and use aren’t standardized or strictly regulated, it’s just a matter of time. AI brings about several legal challenges, ranging from the question of who should be held accountable for the harm caused by the AI system to concerns about the copyright of the AI-generated content. For now, the main issues concern privacy and data protection laws, intellectual property rights, consumer protection regulations, and compliance with anti-discriminatory regulations.
The aim of the Legal and Regulatory Compliance Assessment is to make sure that your system operates within the boundaries of the law. Compliance helps avoid legal violations, penalties, and reputational damage.
Validity and Reliability Assessment
Validity and Reliability Assessment evaluates the accuracy, consistency, and credibility of the AI system’s performance and results. We scrutinize the outputs and performance in validity assessment to ascertain their alignment with the intended objectives and requirements. Conversely, reliability assessment focuses on examining the repeatability and consistency of the outputs, ensuring their ability to produce reliable results consistently.
The purpose of Validity and Reliability Assessment is to ensure that the AI system can be relied upon and to identify any potential limitations or weaknesses to mitigate.
Report and Recommendations
At this stage, we summarize the findings and observations from the AI Tech Due Diligence and provide actionable suggestions or guidance. These recommendations aim to address identified issues, enhance the system’s performance, mitigate risks, and align with best practices. They may cover various aspects, such as data collection and processing, model architecture and optimization, compliance measures, user experience improvements, or ethical considerations.
The recommendations are intended to assist stakeholders in making informed decisions and implementing necessary changes or enhancements to the AI system. This kind of report serves as a guiding light for your system development.
A wrap-up
That’s it for now! We know it’s a lot to take in, but trust us, Technical Analysis in AI Due Diligence is a crucial part of developing any AI system.
We offer all the services mentioned in this article, whether they involve solutions we’ve developed, those from another company, or your internal team. Get in touch with us today to learn more about how we can help you navigate the complexities of AI technology and make sure your systems meet all the technical, legal, and ethical requirements.