They say the only certainty in life is uncertainty, and while this mindset might help us navigate our daily lives, businesses cannot afford to operate in the same manner. They must plan months and even years ahead, which applies especially to the retail sector. Every decision has to be carefully considered to ensure the provision of goods that meet market demand.
As artificial intelligence integrates into various industries, it has also found its place in retail. In today’s article, we will explore how artificial intelligence can be utilized in demand forecasting.
The customer is always right
Satisfying customer needs plays first fiddle in the retail industry; there’s no doubt about that. Their shopping choices directly impact revenue and can even determine the success or failure of a business.
To achieve this, it’s crucial to not only understand what customers want but, most importantly, will want. Without this essential insight, no retail company can effectively strategize for production, warehousing, transportation, and distribution. Anticipating customer needs forms the foundation for making business decisions that align with current demands and position the company to respond proactively to evolving market trends.
Demand forecasting in times gone by
Even in ancient times, traders and merchants used their knowledge and experience to predict customer preferences and seasonal demand. But it was in the early 20th century, with the growth of department stores and more organized retail chains, that demand forecasting became more structured. Retailers started to gather and analyze sales data to make informed decisions about inventory and pricing.
With the introduction of computers and more advanced data analysis techniques, and the rise of the internet, the evolution has continued. The actual game changer was sophisticated forecasting models that incorporated various factors beyond historical sales data, such as economic indicators and market trends.
Today, the advent of big data, advanced analytics, machine learning, and artificial intelligence has revolutionized demand forecasting in retail. These technologies allow retailers to analyze vast amounts of data in real time and make more accurate predictions. Predictive analytics tools have become crucial for understanding customer behavior, optimizing inventory levels, and improving supply chain management.
Retail can’t afford crystal ball gazing
The retail market is influenced by a multitude of factors that contribute to its dynamics and fluctuations. Here are some key factors that come into play in the retail industry:
- Seasonal demand, for example, outdoor equipment in summer and hot beverages in winter
- Consumer trends, for instance, current plant-based eating trend
- Fashion cycles, such as mom jeans vs. skinny jeans in women’s fashion
- Demographic changes, like supplements for seniors in aging populations
- Societal and lifestyle shifts, for example, athleisure trends connected to the rise of remote work
- Economic conditions, such as decreased demand for luxury goods during a recession
- Global events, like shift to e-commerce during the COVID-19 pandemic
- Supply chain disruptions, for example conflict-induced disruption in electronic component manufacturing halting worldwide laptop production
- Regulatory changes, like stricter emissions regulations influencing the car models that can be sold
- Cultural events, as a recent illustration, the release of the “Barbie” movie and the peak in pink item sales.
Analyzing all of these factors simultaneously, along with past events and their potential interconnections, would be impossible without the assistance of artificial intelligence.
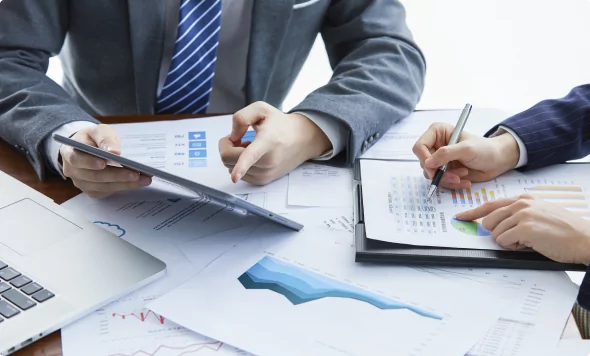
AI-powered predictive analytics: how it works
With AI predictive analytics, you can effectively strategize for production, warehousing, transportation, and distribution. Here are the techniques we use in NeuroSYS:
Data collection
The whole process starts with gathering as much historical data as possible, including sales records, inventory levels, customer behavior, and external factors described above, like holidays, weather, and global events.
Algorithm selection
Then, we select and develop AI algorithms like machine learning models based on the nature of the data, forecasting tasks, and outcomes we want to achieve.
Model training
The next big step is model training. The selected algorithms are trained using historical and current data. In the process, the model learns the relationships and patterns in the data that drive consumer demand fluctuations.
AI training validation
We need to assess the performance and accuracy of the AI models by testing them with a validation dataset. We do it to ensure that the AI models can accurately perform on new data they haven’t been trained on.
Big data analysis
Then, AI algorithms process and combine data from various sources to uncover relationships between the factors and hidden patterns.
Integration
The generated forecasts can be integrated into the retailer’s decision-making processes. This includes optimizing inventory management, supply chain operations, production planning, etc.
Monitoring
Of course, we can’t forget about constant monitoring of the accuracy of forecasts and comparing them to actual demand helps refine and improve the models over time. This feedback loop enhances the accuracy of future predictions.
Wrap-up on AI demand forecasting
AI-powered predictive analytics has evolved into an essential tool for retailers to navigate the complexities of the market, anticipate demand, and optimize their operations. The true power of this approach lies in AI models’ capacity to unearth hidden patterns in real time, a feat that surpasses the capabilities of even the most extensive teams of analysts.
If you’re eager to delve deeper into the realm of AI’s impact on the retail sector, we invite you to reach out to us for further insights and information. Contact us for a free consultation!