Artificial Intelligence (AI) has become a critical tool for businesses, with studies showing that AI can boost profitability by up to 38% across industries. As of 2023, 63% of companies have increased their AI investments, recognizing its potential to enhance decision-making and streamline operations. However, the success of these initiatives depends heavily on one key factor: data readiness. Gartner reports that poor data quality costs organizations an average of $12.9 million annually, underscoring the importance of having clean, well-organized, and accessible data. For CEOs and CIOs, this guide outlines the essential steps to prepare your organization’s data for AI, drawing on proven strategies and industry insights to ensure effective implementation.
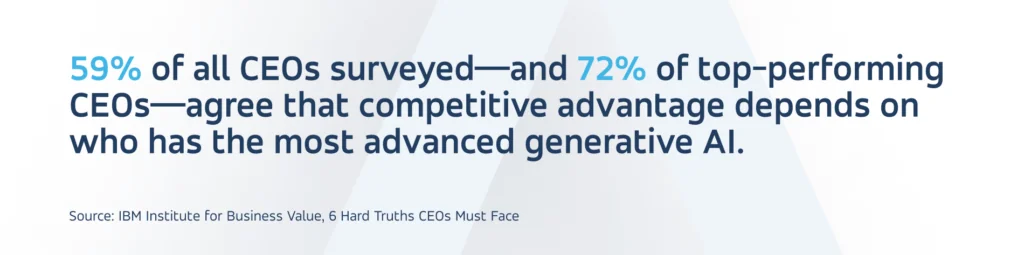
Assessing Your Data Landscape
Before you can effectively deploy AI, it’s essential to understand your current data environment. Many companies struggle with data silos, where critical information is isolated across different departments. A study by IDC found that only 10% of businesses have a modern data infrastructure capable of seamless integration and analysis. Centralizing your data through a data warehouse or data lake is a practical first step, ensuring that your data is consistent, accessible, and ready for AI applications.
AI-Ready Data Preparation Rubric to Level Up Towards Data Readiness & AI Adoption
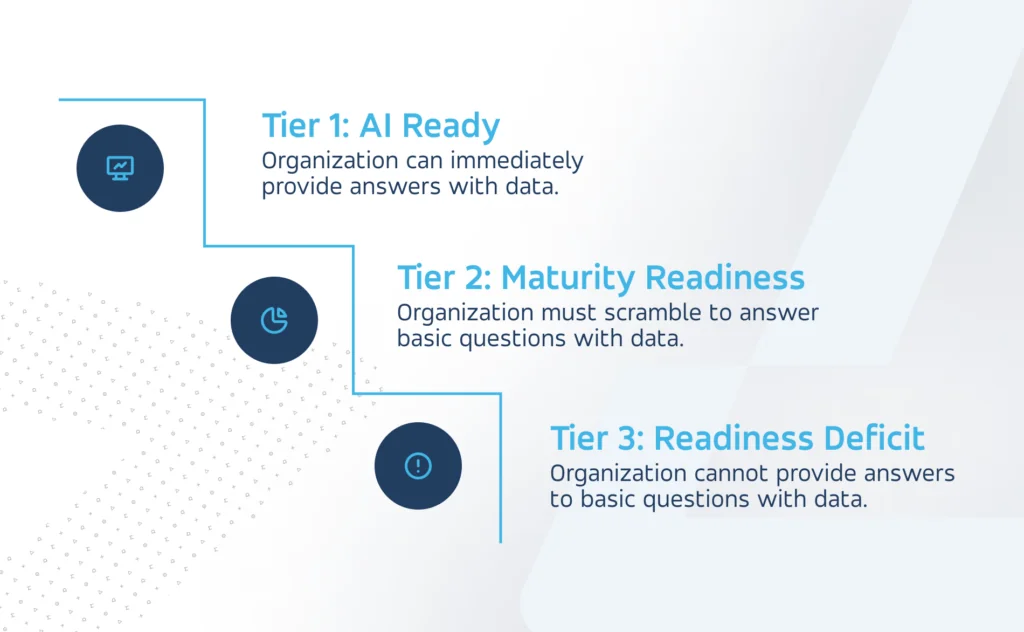
Addressing Data Challenges in AI Implementation
Implementing AI involves several challenges related to data:
- Data Quality: Ensuring data is accurate and free from bias is essential. Poor-quality data can lead to unreliable AI models and costly errors.
- Scalability: As data volumes grow, your infrastructure must be able to scale accordingly. This ensures that AI models can process large datasets efficiently without compromising performance.
- Integration of Diverse Data Sources: AI often requires data from multiple sources, which can be challenging to integrate due to differences in format and structure. Effective integration is crucial for accurate AI outcomes.
- Data Privacy and Compliance: As AI systems handle sensitive data, ensuring compliance with regulations like GDPR and CCPA is vital. A robust governance framework is necessary to avoid penalties and maintain trust.
Read also: The most effective big data tools and techniques
Steps to AI-Ready Data
Once your data is centralized, the preparation process involves several key steps:
- Data Cleansing
- Initial Review: Examine field names and descriptions to identify any anomalies or missing elements.
- Variable Check: Assess the range, uniqueness, and health of variables within your dataset.
- Anomaly Detection: Identify and evaluate unusual data points using visual tools (e.g., histograms, scatter plots) or statistical methods.
- Pattern Exploration: Investigate patterns and relationships in the data to uncover significant insights.
Cleaning your data is crucial to eliminate errors and inconsistencies. According to Alteryx, 20% of IT and data leaders identify poor data quality as a significant challenge. Automated tools can streamline this process, reducing the time spent on manual corrections and allowing your team to focus on strategic initiatives.
- Duplicate Removal: Clear out redundant records to optimize data storage and ensure accuracy.
- Relevance Filtering: Remove irrelevant data entries that do not contribute to the AI model’s objectives.
- Missing Data Handling: Address gaps in the data by investigating and filling in missing values.
- Outlier Management: Adjust or eliminate outliers that could distort the analysis.
- Structural Correction: Fix structural issues such as formatting inconsistencies and typographical errors.
- Quality Validation: Ensure data consistency and integrity by thoroughly documenting the purification process.
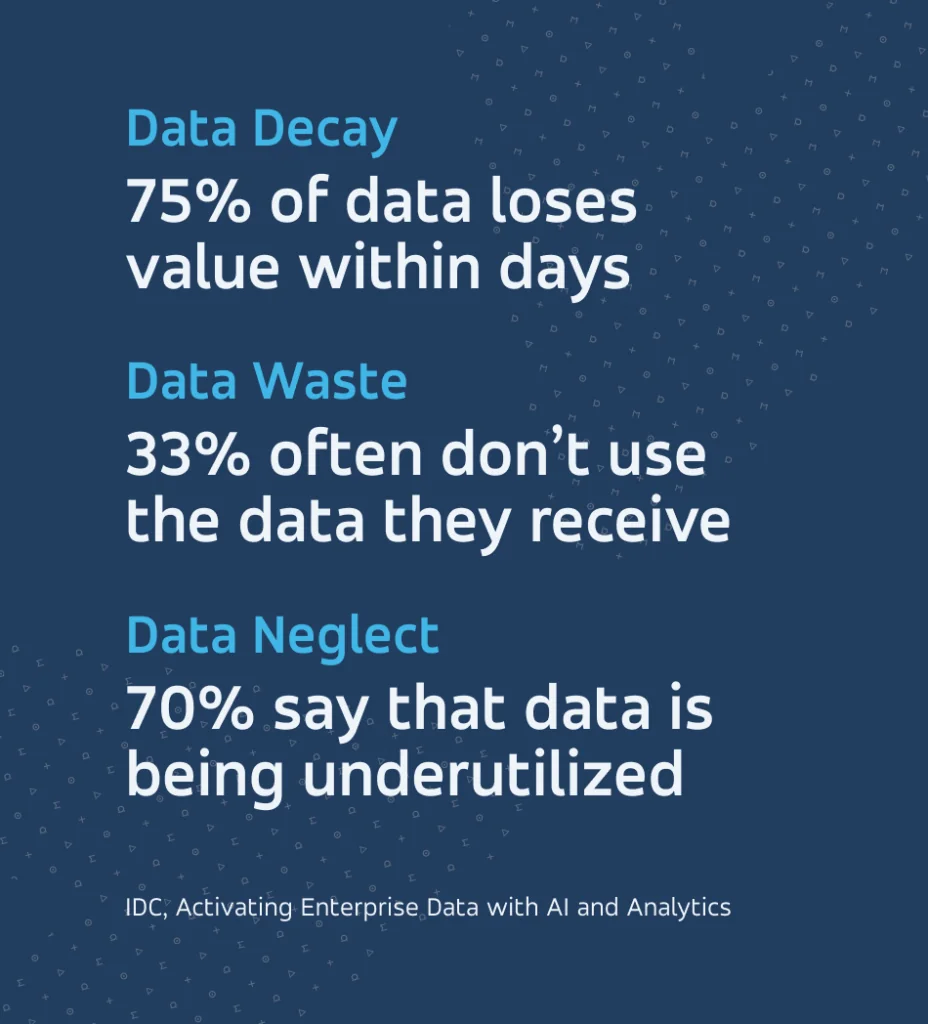
- Data Integration
AI projects often require data from multiple sources. Effective data blending combines these datasets to provide a comprehensive view, which is essential for accurate analysis. Modern tools simplify this process, ensuring that data is
- Source Aggregation: Collect and prepare datasets from various sources, ensuring they are aligned in format and structure.
- Dataset Fusion: Seamlessly combine datasets, ensuring compatibility and coherence.
- Result Verification: Validate the integrated datasets for consistency and resolve any discrepancies.
- Data Profiling and Validation
Before data is fed into AI models, it must be rigorously checked for consistency and relevance. Automated profiling tools help ensure that only high-quality data is used, reducing the risk of inaccurate predictions
- Structural Analysis: Review dataset size and type to confirm proper formatting and compatibility for intended use.
- Content Verification: Conduct checks for gaps, errors, and systemic issues within the data.
- Relationship Mapping: Identify and document connections between data entities, ensuring these links are maintained during data migration.
Read also: When Generative AI Isn’t the Right Choice for Your Business: An Expert’s Take on Gartner’s Insights
- Data Orchestration (ETL: Extract, Transform, Load)
ETL processes are fundamental in preparing data for AI. This involves extracting data from various sources, transforming it into the correct format, and loading it into a central repository. Ensuring your ETL processes
- Data Extraction: Pull data from multiple sources, confirming its quality, especially from legacy systems.
- Transformation: Thoroughly clean and format the data to meet the technical requirements of the target environment.
- Loading: Store the transformed data in a centralized repository, followed by quality checks to ensure readiness for analysis.
- Data Wrangling
Even after cleansing and blending, data may require additional processing to meet AI model requirements. Data wrangling involves further
- Continuous Exploration: Re-examine data during analysis to resolve issues affecting model performance.
- Structural Adjustment: Align the data structure with the needs of your analytical model for optimal results.
- Ongoing Cleansing and Enrichment: Continue correcting errors, removing duplicates, and integrating additional authoritative data sources.
- Process Documentation: Save and document the refinement processes to ensure they can be reproduced for future projects.
These steps will help you transform raw data into AI-ready data, setting the foundation for successful AI deployment and enabling your organization to fully leverage AI’s capabilities.
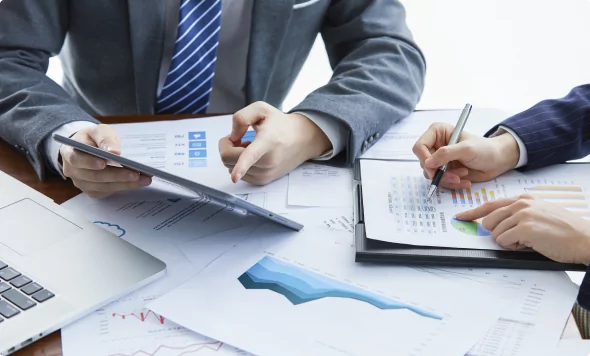
Implementing a Data Catalog for AI-Readiness
A data catalog is an effective tool for managing and utilizing AI-ready data. It provides a comprehensive inventory of your data assets, complete with detailed metadata and context. This ensures that data scientists and analysts can quickly access the right datasets, enhancing efficiency and accuracy in AI projects. A modern data catalog also supports data governance, helping to maintain compliance with regulations and improve overall data management.
Addressing Data Readiness on a People and Strategy Level
Successfully preparing your data for AI implementation is not just a technical challenge but also a strategic one that involves your people and organizational culture. For AI to be effectively integrated into your operations, it is crucial to align your workforce and leadership around clear data strategies and practices. Here’s how to approach data readiness from a people and strategy perspective:
Building a Data-Centric Culture
A critical aspect of AI readiness is fostering a data-centric culture within your organization. This means ensuring that all employees, from top management to frontline workers, understand the value of data and their role in maintaining its quality and accessibility. A study by Deloitte found that only 35% of Chief Data Officers (CDOs) feel they have sufficient resources to manage data effectively, indicating a significant gap in organizational readiness.
To build a data-centric culture:
- Leadership Commitment: Your leadership team must actively promote and model the importance of data quality and accessibility. This involves setting clear expectations about data usage and investing in tools and training that support data literacy across the organization.
- Data Literacy Programs: Implement ongoing training programs to improve data literacy across all levels of the organization. Employees should understand the basics of data governance, how to handle data responsibly, and the implications of poor data quality on AI outcomes.
- Incentivizing Data Stewardship: Encourage employees to take ownership of the data they handle by integrating data stewardship responsibilities into performance evaluations and incentives.
Establishing a Clear Data Strategy
For AI initiatives to succeed, your organization needs a clear and comprehensive data strategy that aligns with your overall business goals. This strategy should address how data is collected, managed, and utilized across the company. It should also define the roles and responsibilities related to data management, ensuring that everyone knows their part in maintaining AI-ready data.
Key elements of a data strategy include:
- Data Governance Framework: Implement a robust data governance framework that defines how data is stored, accessed, and shared. This framework should include policies for data privacy and security, ensuring compliance with regulations like GDPR and CCPA.
- Stakeholder Engagement: Successful AI implementation requires collaboration across multiple departments. Develop a stakeholder engagement plan that connects key players – uch as data engineers, data scientists, and business analysts – ensuring that data initiatives are aligned with business objectives and that data is used effectively across the organization.
- Data Quality Assurance: Establish processes for continuous data quality monitoring and improvement. Regular audits and the use of automated tools can help maintain high data standards, reducing the risk of poor data compromising AI models.
Empowering Data Leadership
The role of data leadership, particularly the CDO or equivalent, is crucial in guiding your organization towards AI readiness. The CDO should be empowered to drive data initiatives, enforce data governance policies, and advocate for the necessary resources and tools. As AI technologies evolve, the CDO’s role will become increasingly strategic, requiring a focus on both the technical and ethical aspects of data management.
Actions to empower data leadership include:
- Direct Access to C-Suite: Ensure that the CDO has direct access to the CEO and other C-suite executives to influence decision-making and secure the necessary support for data initiatives.
- Cross-Functional Collaboration: Facilitate regular cross-functional meetings where the CDO can collaborate with other executives, ensuring that data strategies are integrated into broader business strategies.
- Investment in Data Capabilities: Support the CDO by investing in advanced data management tools and technologies that enhance the organization’s ability to handle large datasets, perform complex analyses, and maintain data quality.
Developing a Successful Proof of Concept (PoC)
A Proof of Concept (PoC) is a crucial step in the AI implementation process, allowing organizations to test AI applications in a controlled environment before scaling them up. A well-executed PoC provides valuable insights, minimizes risks, and helps refine your AI strategy. If you want to do a simple AI PoC, you can do the integration manually, which will reduce your initial costs. You don’t have to start a data warehouse project. Here are the essential steps to developing a successful PoC:
- Define the Problem and Objectives
Start by clearly defining the problem or opportunity that AI will address. It’s crucial to set specific, measurable objectives for the PoC, ensuring that it aligns with your broader business goals. Consider where AI can deliver the most value and focus on those areas first.
- Assemble the Right Data
Locate and centralize the data needed for the PoC. This may involve gathering data from various departments or external sources. Ensure that the data is of high quality—clean, consistent, and well-labeled. This step is foundational, as the success of the AI model will heavily depend on the quality of the data used.
- Evaluate for Business Value
Assess the potential business value of the PoC. This includes evaluating the cost, risk, and expected benefits. Consider the technical feasibility as well as the availability of in-house expertise. If necessary, seek external support from data science experts or AI service providers to ensure the PoC is designed for success.
- Design and Deploy the Solution
Develop the AI model and infrastructure needed for the PoC. Use a test-and-learn approach, iteratively refining the model based on feedback and results. Ensure that the solution is scalable and compatible with your existing systems. Key technologies to consider include hardware infrastructure, AI frameworks, and visualization tools.
- Define Evaluation Criteria
Establish clear criteria for evaluating the success of the PoC. This should include technical metrics like accuracy, completeness, and timeliness, as well as business metrics such as ROI and customer impact. Evaluate the AI model’s performance in terms of fairness, transparency, and safety to ensure it meets ethical standards.
- Scale Up and Optimize
Once the PoC has demonstrated value, begin scaling the AI solution across the organization. This involves optimizing the model, expanding its use to other business areas, and integrating it with existing workflows. Continuously monitor performance and make adjustments as needed to maximize the impact of the AI solution.
Conclusion
Preparing your data for AI is a multifaceted process that involves more than just technology; it requires strategic planning, leadership, and a strong data-centric culture. For CEOs and CIOs, overseeing this process is crucial to ensuring that your organization’s data is clean, centralized, and effectively managed. By following the steps outlined, assessing your data landscape, addressing data readiness on a people and strategy level, implementing a data catalog, and developing a robust PoC, you can lay a solid foundation for AI success. With the right approach, AI can transform your business, driving innovation, efficiency, and growth in a competitive market.
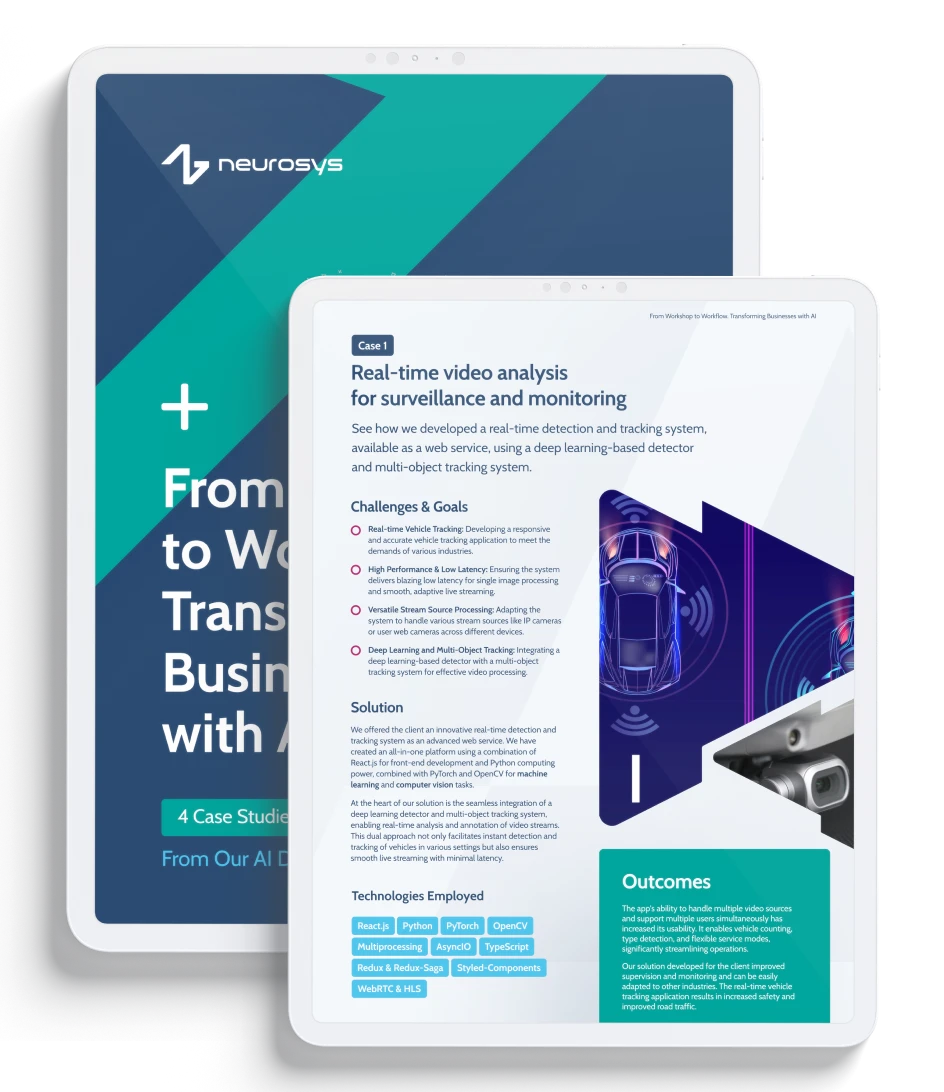