Generative AI (GenAI) has seen rapid adoption across enterprises, promising revolutionary changes in various business operations. However, its allure can often lead to misapplication, resulting in high failure rates and wasted resources.
To navigate this landscape effectively, business leaders must understand when GenAI is not the ideal tool for their use case. For example, a large financial institution might hesitate to use GenAI for analyzing sensitive customer data because it may involve sharing internal information with external systems, raising significant concerns about data privacy and security.
Similarly, a major multinational corporation seeking to automate complex strategic decision-making processes might find that GenAI falls short in delivering reliable insights because it cannot fully grasp the intricate market dynamics and nuanced context of global operations.
This summary of Gartner’s insights will guide you through critical considerations and provide a framework for evaluating the applicability of GenAI within your organization.
Key Findings
Understanding When Not to Apply GenAI
Many IT leaders struggle to discern when GenAI is appropriate for their use cases. The hype surrounding GenAI can lead to its application in unsuitable scenarios, increasing project complexity and failure risks.
Focusing solely on GenAI can overshadow other AI techniques that might be more suitable, thereby diminishing potential business value.
AI Techniques Are Not Mutually Exclusive:
Combining different AI techniques often results in stronger systems with better accuracy, transparency, and performance while reducing costs and data requirements.
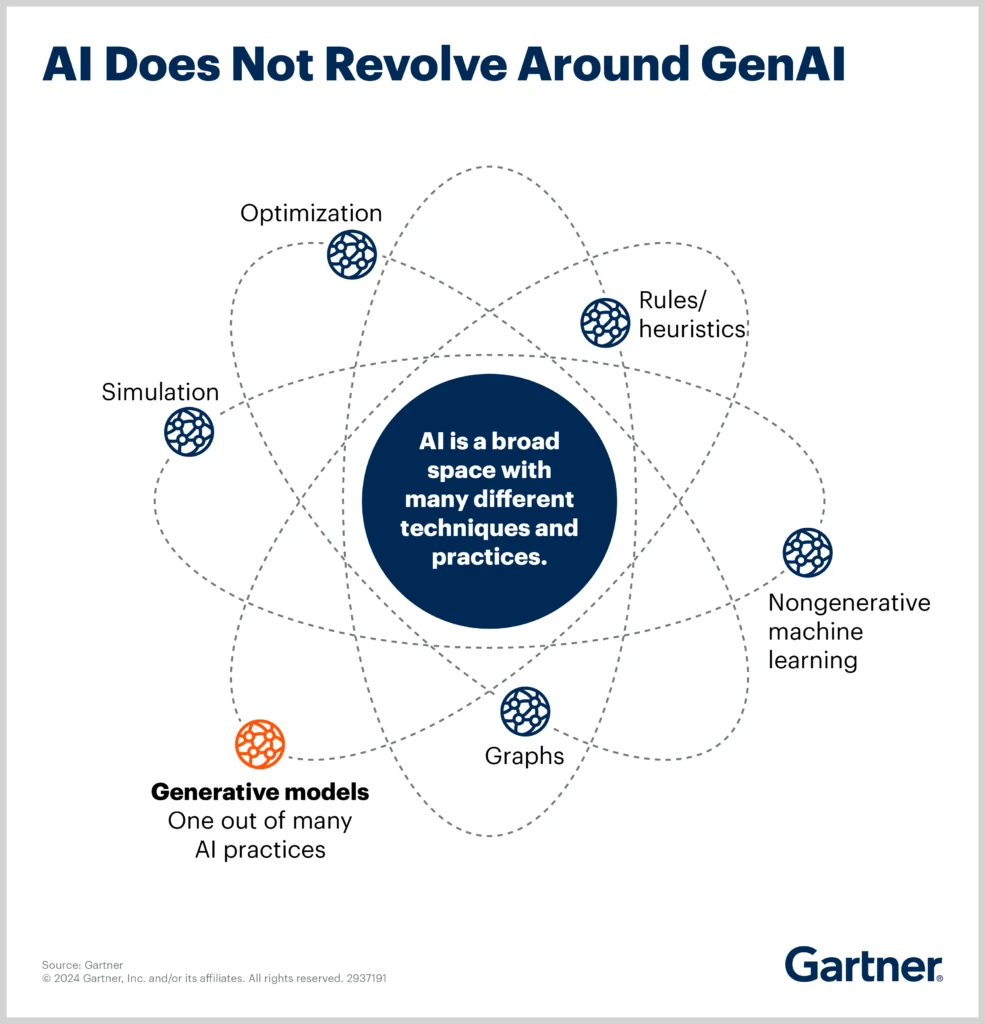
Recommendation: An Approach to Use Case Hunting
Categorize and Evaluate Use Cases
Systematically categorize each use case to evaluate the feasibility of GenAI. Use cases involving prediction and forecasting, planning and optimization, decision intelligence, and autonomous systems are generally not well-suited for GenAI in isolation.
Utilize Alternative AI Techniques
When GenAI is not the right fit, consider other AI techniques such as non-generative machine learning (ML), optimization, simulation, rule-based systems, and graphs. These alternatives often offer more reliability and a better-understood approach.
Additionally, in some cases, non-AI solutions such as traditional software tools or specialized business systems might be more appropriate and cost-effective, particularly when they align well with the specific needs and constraints of the organization. Balancing AI with proven non-AI methods can help ensure that businesses use the most efficient and economical tools for their unique challenges.
Combine AI Techniques
Combining GenAI with other AI techniques can mitigate some of its limitations, such as inaccuracies and hallucinations. Conversely, GenAI can support other AI techniques, for instance, by serving as a natural language interface to other systems.
Evaluation of GenAI Usefulness Across Use-Case Families
Gartner’s research divides AI use cases into 12 families, each evaluated for the relative usefulness of GenAI models. This categorization helps determine when GenAI might be misapplied:
Low Usefulness
- Prediction/Forecasting – Use cases like risk prediction, customer churn prediction, and sales/demand forecasting are not well-suited for GenAI. These tasks require numerical predictive and statistical modeling that current GenAI models are not designed to handle.
- Planning – Planning and optimization use cases, such as inventory optimization and route planning, require precise calculations, which are not the strength of generative models.
- Decision Intelligence – Decision support and automation tasks need reliable outputs and explainability, which GenAI lacks. Critical decisions, such as HR or financial planning, should not rely on GenAI outputs.
- Autonomous Systems – Current GenAI models are not robust enough for autonomous applications like self-driving cars or advanced robotics, as they require close human supervision.
Medium Usefulness
- Segmentation/Classification – Clustering and object classification can benefit from GenAI but often require additional techniques for optimal performance.
- Recommendation Systems – GenAI can enhance recommendation engines and personalized advice systems, although other AI techniques might also be necessary.
- Perception – Object detection and recognition tasks can use GenAI, but with the need for robust accuracy provided by supplementary methods.
- Intelligent Automation – Tasks like intelligent document processing and robotic process automation can leverage GenAI, though integration with other systems is beneficial.
- Anomaly Detection/Monitoring – Abnormal transaction detection and monitoring can use GenAI but might be better served by more established AI techniques.
High Usefulness
- Content Generation – Text, image, and video generation, as well as synthetic data creation, are prime areas where GenAI excels.
- Conversational User Interfaces – Virtual assistants and chatbots benefit significantly from GenAI’s capabilities.
- Knowledge Discovery – Searching and mining knowledge bases are well-suited to GenAI applications.
Alternative AI Techniques
Given that GenAI is not always the best fit, consider these alternative AI techniques:
- Nongenerative Machine Learning – Ideal for category predictions and regression tasks, such as customer segmentation, anomaly detection, and predictive maintenance.
- Optimization – Useful for planning tasks, such as inventory management, scheduling, and route planning, where it maximizes benefits by managing trade-offs.
- Simulation – Supports decision making by testing scenarios virtually, applicable in financial modeling, strategic analysis, and process simulations.
- Rules/Heuristics – Captures expert knowledge for decision making, suitable for fraud detection, loan approval, and medical diagnosis where explainability is crucial.
- Graphs – Knowledge graphs are better for tasks requiring explainability and precise data retrieval, such as recommendation systems and fraud detection.
Combining GenAI with Other Techniques
Combining GenAI with other AI techniques can enhance system robustness and functionality:
- Nongenerative ML and GenAI – Use pretrained GenAI models to build classifier models for specific applications, or generate synthetic data for training purposes.
- Optimization and GenAI – Enhance enterprise search by making its interface more conversational and improving accuracy.
- Simulation and GenAI – Accelerate simulations by substituting parts with GenAI models, allowing more extensive and faster simulations.
- Graphs and GenAI – Extract entities and relationships from unstructured text to populate a graph database, improving knowledge management capabilities.
- Rule-based Systems and GenAI – Enhance chatbots and robo-advisors with a mix of rule-based techniques and generative models, providing sophisticated decision-making support.
Conclusion
While GenAI offers groundbreaking possibilities, it is not a one-size-fits-all solution. Business leaders must carefully evaluate their use cases to determine when GenAI is appropriate and when to consider alternative AI techniques. By systematically categorizing use cases and exploring combinations of AI techniques, organizations can build robust, efficient, and effective AI systems that drive real business value. Following Gartner’s insights will help ensure that your AI projects succeed and deliver the expected outcomes.
If you lack expert knowledge on possible use cases of GenAI in your company or you need AI tools, sign up for a 1h free consultation. Our expert will introduce you to the details of the possibilities of using artificial intelligence and its tools in your company.
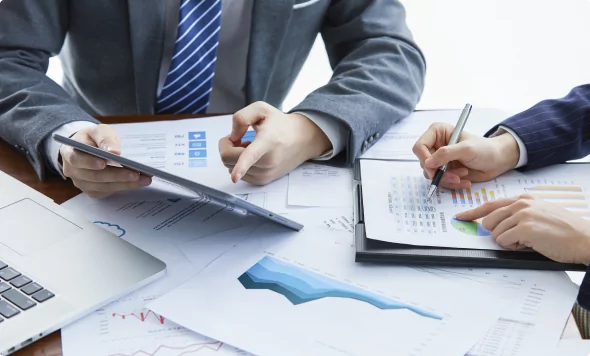