The clash of two worlds, artificial intelligence and pharma, revolutionized the latter. What used to look like a pipe dream or scenario straight from sci-fi movies, now is increasingly used in real-life applications.
Artificial intelligence, one of the fastest-growing technologies of today (and tomorrow!), is changing the face of modern pharma. However, AI, with its specialized branches, like machine learning (ML) and computer science, are no beginners in the widely understood medical field. Precisely applied algorithms for cancer diagnosis, manage health information, match patients with doctors, and many more already exist.
Pharmaceutical challenges
The medical subdomain we focus on in this article, covering medicinal drugs development and manufacturing, owes much to the latest technologies. Before a medication can be marketed, great efforts must be made beforehand. Once the promising compounds are determined for trials, scientists conduct tests on the pharmacodynamics (how the substance affects functions of the body) and pharmacokinetics (what is the substances’ impact on bodily processes). In the case of traditional research and development of new medicines, the approximate time for a new drug to make its way from the discovery to entering the market takes on average 10 years, costing over $2 billion. A substantial part of overall costs is necessary failures, as among vast amounts of substances only a small percentage will eventually be authorized for use.
Artificial intelligence is applied in the healthcare industry due to algorithms’ potential to mimic human-like cognitive functions in response to surroundings. AI predicts certain outcomes based on processed data on existing items and their properties. Employing machine learning arms scientists with powerful algorithms, capable of carrying out processes based on large datasets, inevitable in research within the medical field. Machine learning plays an important role in preliminary, early-stage drug discovery, through processing datasets and analyses necessary for further steps.
Take a look at how algorithms are changing the pharmaceutical industry in a world that can’t wait for new treatments and solutions to revolutionize healthcare.
3 real-life use cases of AI/ML applications in the pharmaceutical industry
Drug discovery and development
The application of AI and ML leads to significant savings in the costly process of pharmaceutical research and development. Algorithms allow shortening of the drug discovery process, leading to faster rejection of dead-end paths in medicinal research.
For example, Exscientia, a British pharmaceutical company, developed a drug (known under the working name DSP-1181) using an algorithm. The formula is meant to aid patients with obsessive-compulsive disorder and is the third AI-powered research project of the company. According to the company’s statement, employing AI shortened research from several years to 12 months [1].
Another example of employing algorithms in drug discovery is the AI-powered research conducted at MIT focused on Escherichia coli bacteria, based on data analysis of over 2,000 growth-inhibiting particles. What followed was a molecular analysis of existing drugs performed by algorithms, the result of which was a selection of 23 structures for further testing. The research allowed scientists to pick an effective compound, halicin, capable of killing antibiotic-resistant bacteria. Scientists engaged in the works believe that, aside from achieving the set goal, the “side effects” of this particular research can result in the development of several other remedies based on conclusions on bactericidal chemical structures discovered by applied algorithms [2].
Drug screening
Algorithms support medicine development by assessing the properties of substances subjected to testing. AI predicts physical properties, bioactivity, and toxicity in a far more efficient and accurate way than traditional methods. The screening involves using high-throughput libraries, rapid tests of thousands to millions of compounds assessed for biological activity, of which particular substances cost usually up to $100. Traditionally executed screening not only costs several millions of dollars, but also takes months of work. Adopting artificial intelligence in the process shortens the screening time, as even billions of compounds can be examined within days [3].
Drug design
During a structure-based drug development process, one of the vital elements is examining the 3D structure of the selected protein. The process can be performed by e.g. homology modeling (comparative protein modeling, constructing a model of the protein, illustrating its features in an atomic-resolution figure), but usage of artificial intelligence powers the process to achieve a new quality of drug design. Utilizing deep neural networks (DNN), AI predicts protein properties and presents a score used to assess the precision of the examined 3D protein model. As a result, artificial intelligence tools, like Google’s Deepmind protein library, can find structure models matching predictions, leading to faster drug development [4].
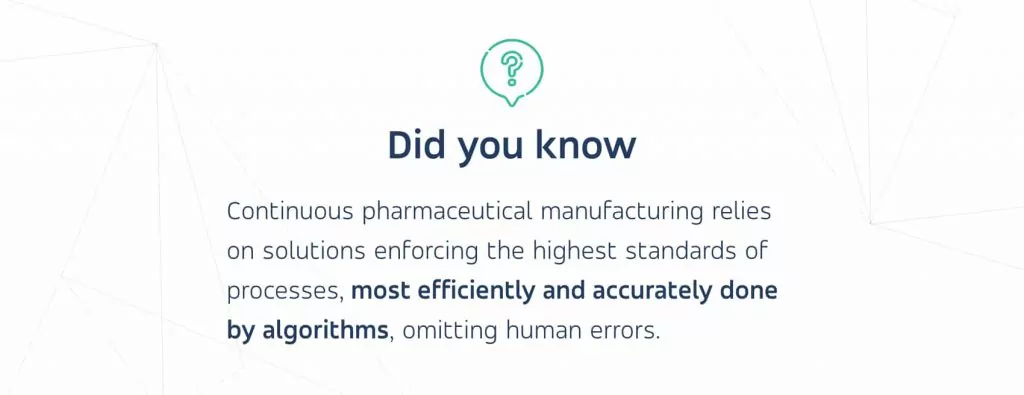
Drug manufacturing
Automated chemical synthesis
Automation in the pharmaceutical industry increases reliability and reproducibility, presenting benefits similar to other industries, e.g. reducing the valuable expert time spent on tiresome, repetitive tasks. Contrary to previously used traditional methods, enabling a smaller range of focus on tested substances, AI-powered processes enable complex, multistep synthesis of intricate compounds for their more efficient, and faster production.
Automated microbiological analysis
At NeuroSYS, deep learning algorithms were employed to support bacterial colonies’ detection in examining images of Petri dishes. The solution, aimed at improving the accuracy of automated microbiological analysis, recognized whether the assessed samples remained free from bacteria (negative sample) or bacteria appeared on examined dishes (positive sample). Analysis of sample images was performed without human specialist supervision. The solution is applicable in the pharma industry and industrial microbiology, accelerating the process and minimizing the risk of human errors, previously leading to false-positive results. Furthermore, employing deep learning algorithms enabled avoiding unnecessarily costly multispectral cameras, improving the projects’ cost efficiency.
Drug repurposing
Algorithms can also find new paths for already known solutions. Prediction of interactions between medicines and proteins is the key to successful therapy. Using AI in drug repurposing enables faster prediction of drug-protein interactions and finding new usages, qualifying the treatment in question directly to Phase II of clinical trials, omitting Preclinical Phase (testing on non-human subjects), Phase 0 (testing pharmacokinetics, e.g. oral bioavailability), Phase I (dose-ranging clinical trials on volunteers). Successful repurposing not only shortens development time, reduces financial expenses, but also indirectly contributes to reducing the exploitation of animals in the pharmaceutical industry.
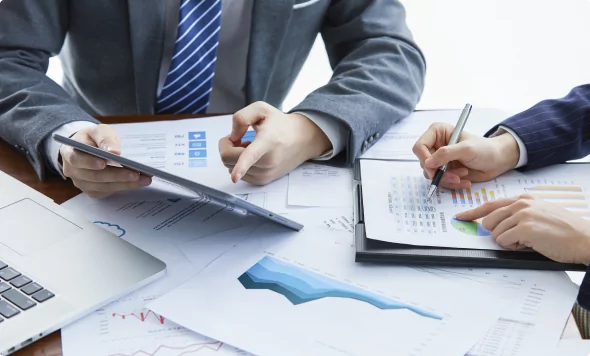
AI supporting hardware in the pharmaceutical industry
Smart devices
The pharmaceutical industry welcomes the Internet of Things with open arms. Usage of smart wearables fosters the use of miniature biosensors, supporting research in the medical field. Smart devices clear the way for gathering large amounts of data for further processing in e.g. drug efficiency analysis and improving patients’ commitment to following medical prescriptions, all in agreement with the data safety policy. In a wider approach, wearables support recognition of health issues and shortening of hospital stays by extending in-home treatment.
Preventive maintenance
There is no large-scale drug manufacturing without automation and specialized machines, working often 24/7 to meet the market demands. Similarly to other industrial branches, the pharmaceutical industry aims to avoid downtimes and unplanned shutdowns caused by equipment failures. Algorithms come to the rescue, gathering vital data via dedicated sensors, constantly monitoring components, mechanisms, and their damage. Incorporating e.g. AR solutions enables performance optimization, integration of control systems, monitoring crucial parameters, and performing maintenance without production line stoppages.
Near future
Despite occasional opinions that AI/ML adoption may be happening too fast, recognized authorities consider AI/ML the future of the medical field, with pharmaceuticals being no exception. The McKinsey report forecasts that algorithm and data-powered solutions can result in $100 billion worth annually, resulting from precise tools for research and development in the field. The use of AI/ML in healthcare creates new opportunities for more efficient, safer and quicker processes aimed at improving health and wellbeing. Verification of properly executed chemical transformations, total elimination of impurities, constant monitoring of right compound proportions, and adequate packaging of particular medications in the right dosages can all be carried out under the watchful eye of AI.
The future of AI/ML in the pharmaceutical industry will most likely involve further development of tools and pharmaceutical process automation software specialized for tasks like laboratory processes automation, property predictions, molecular generation, chemical synthesis procedures, and molecule complexity evaluation. Using algorithms not only frees staff’s hands but offers new possibilities to the industry, increasingly adopting most recent technologies.
Want to stay ahead of your competition? Book your free consultation and see how can AI power your operation!
Sources
[1] Clinical Trials Arena
[2] Massachusetts Institute of Technology
[3] Forbes
[4] Nature