Machine learning’s ability to process massive data and identify complex patterns is of great importance when it comes to solving challenging problems of LifeScience and Pharma, where data and accuracy is the key. In this blog post, we want to take a closer look at real-life examples of successful machine learning solutions for Pharma and LifeScience companies.
Lab Automation
Automation in labs is nothing new since the introduction of robotics decades ago. However, AI adoption brings laboratory robotics to the new level by eliminating or reducing the need for human control over the processes, easily scaling operations and eventually simplifying the work behind complex biological tests to a few mouse clicks.
One of the examples of machine learning application in labs is plate reading with the help of computer vision for more accurate and faster microbiological analysis. Machine learning algorithms can classify and identify the number of bacterial cultures grown on a Petri dish without the need to involve a lab specialist.
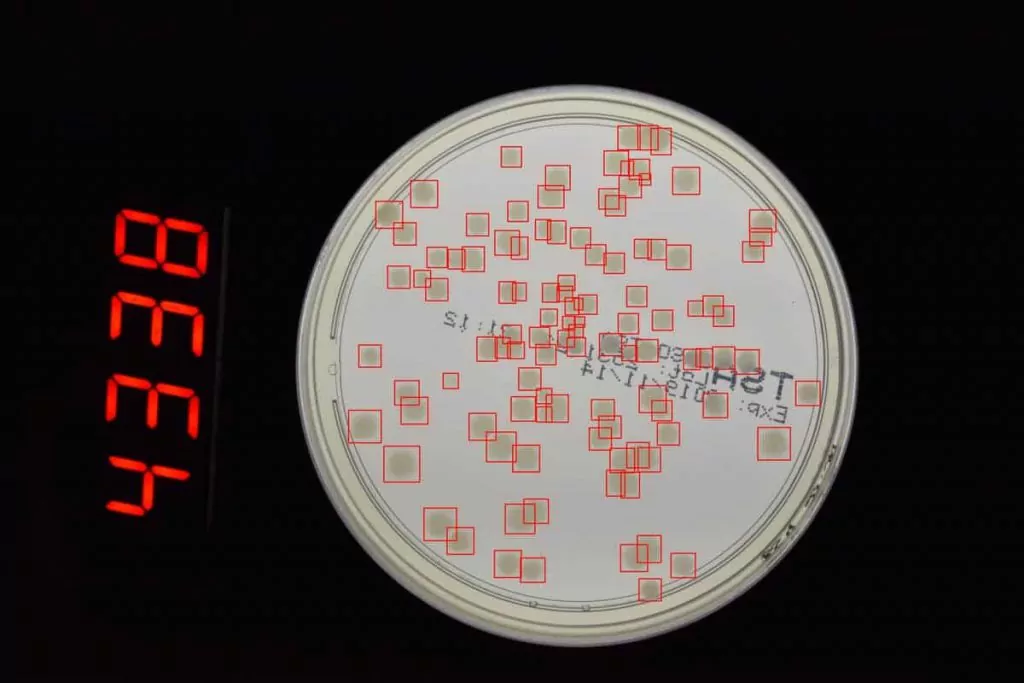
Our R&D team is currently working on the plate reading solution for industrial labs. In cooperation with microbiology experts, we are preparing a database of carefully described photos of Petri dishes with specific species of bacteria. At the moment we have a database of over 10.000 images, which allows us to accurately classify and count the most common types of bacteria in Pharma. As we plan to gather 20.000 images we will continue to work on widening the range of bacterial species that our solution can recognize.
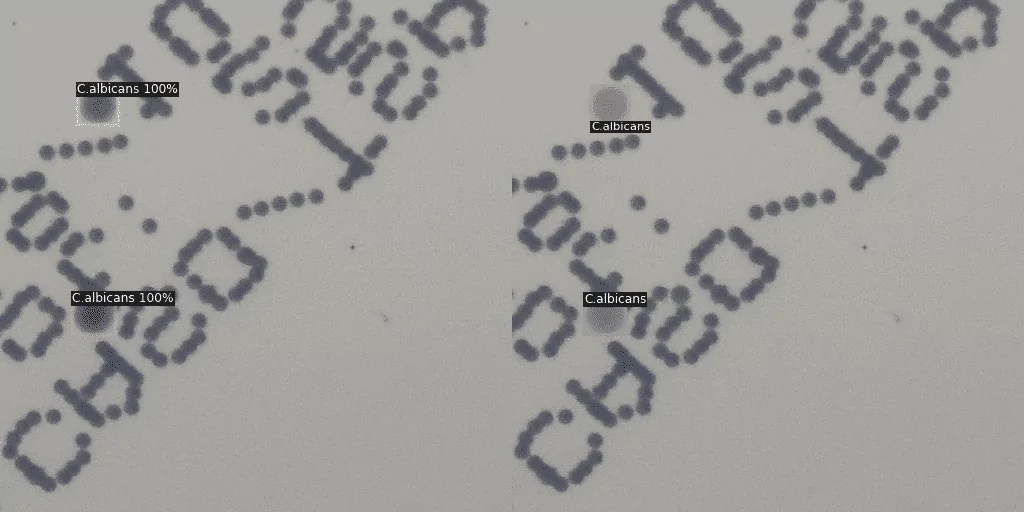
You can read more about the project here or become the early adopter by filling up this contact form.
Assisted Diagnosis & Personalised Treatment
Due to strict regulations in medicine, fully AI-powered diagnostics and ‘robodocs’ still belong to sci-fi plots. However, machine learning algorithms already help in analytics and diagnoses establishment, as well as treatment schemes design.
One of the biggest players in this area is IBM Watson Genomics from Quest Diagnostics which uses machine learning and Big Data to uncover personalised cancer treatment options. The system compares a patient’s genome sequence with massive amounts of information from clinical, scientific and pharmacological databases in order to pick the best matching therapy for particular tumour mutations of the patient. The results are then reviewed by a pathologist before being sent to the patient or relevant doctor.
Another example here is a UK-based company Kheiron Medical Technologies that utilizes computer vision to support breast screening and determine breast cancer earlier. Their solution analyses mammogram images (which are often hard to read by a human eye, and thus prone to false analysis results) and provides radiologists with necessary data to reach a diagnosis faster and more accurately.
A similar approach, but at a wider scope, is taken by Wrocław-based Cancer Center. With the help of machine learning the team at Cancer Center developed a dedicated algorithm that analyzes medical images for oncology and radiology for faster and better than human accuracy.
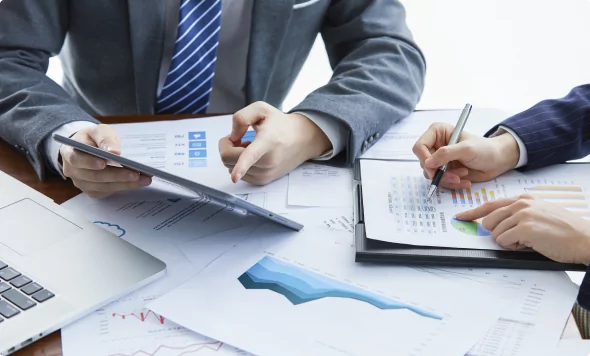
Drug Discovery & Manufacture
It’s not a secret that drug discovery is enormously expensive and time-consuming due to highly qualified specialists needed to be involved and long-running clinical trials that must be performed on a wide scale. Machine learning potential promises to make this process cheaper, faster and more effective.
IBM Watson is one of the leaders in this field with its cognitive platform for identifying new drugs and repurposing existing ones, used by Pfizer for immuno-oncology drugs search. Other top pharma companies (Roche, Sanofi) also cooperate or establish their own subsidiaries that utilize AI algorithms for pharmaceutical research.
Besides traditional pharma giants, due to the big pie at stake, in recent years the market got oversaturated with drug discovery startups. One of the prominent ones is a Canadian company BenchSci with its AI-assisted antibody selection solution, that accelerates the drug discovery process by providing researchers with data about antibody usage. Users can perform a search by easily entering a query (protein, gene, or clone ID) in the system’s search bar. The application then displays links to all the information about antibodies that have been proven to work with the possibility to apply various filters to them.
Reportedly, the BenchSci’s machine learning model that lies in the base of their solution has been trained on 5.1 million antibody products, over 3 million vendor-supplied figures, and 45,000 figures from third-party validators. The data was cross-referenced with over 9 million scientific publications.
The BenchSci’s solution is being used by such companies as Janssen, Pfizer, GSK etc. They also offer free access for students and researchers at academic, government, and nonprofit institutions.
* * *
We hope you enjoyed reading. To learn more about the commercial application of AI and machine learning keep an eye on our R&D teams’ findings or get in touch with us for more tailored recommendations.