You may have heard the age-old saying, ‘Better safe than sorry,’ and it’s not just applicable to everyday situations but also business endeavors. That’s where predictive maintenance comes into play, widely employed across industries such as manufacturing, automotive, and other machinery-heavy or high-risk sectors.
The core idea behind it is to proactively anticipate and address potential equipment failures before they result in costly breakdowns. By adopting this approach, manufacturers can significantly enhance operational efficiency while reducing overall expenses. So, the question arises: how can this strategy be successfully implemented?
Prevention is better than cure
Manufacturing downtime can indeed be quite costly and impact your revenue directly. When production comes to a halt, it results in the absence of finished products and means paying for your employees’ idle time.
Moreover, inactivity in one part of the manufacturing process can create bottlenecks that reverberate throughout the entire production chain, affecting other interconnected operations. The consequences of such downtimes can be severe, leading to delays in meeting production deadlines and potentially resulting in penalties or contract breaches.
Additionally, emergency repairs that arise from unexpected breakdowns can be more expensive and time-consuming than regular maintenance.
It is evident that, in this scenario, an ounce of prevention is worth a pound of cure. That’s where preventive maintenance comes into play. By proactively identifying potential issues in advance, preventive maintenance allows for scheduled service or repairs to be carried out before any major breakdown occurs.
How does AI-based preventive maintenance work?
First, there are sensors
You might be curious about how a system can keep track of what’s happening with the machinery. Well, the answer lies in sensors. Depending on the specific equipment being monitored, preventive maintenance relies on various types of sensors. Some of the common types include:
- temperature sensors
- humidity sensors
- vibration sensors
- infrared sensors
- pressure sensors
- flow sensors
- acoustic sensors
- sonic sensors.
Sensors play a crucial role in AI-based predictive maintenance as they collect real-time data from equipment and machinery. These essential devices can detect a wide range of information, such as abnormal temperature, potential misalignment of parts, or insufficient machine lubrication. In many cases, they surpass human capabilities in sensitivity and precision, enabling them to perceive details imperceptible to human sight or hearing.
The data gathered by these sensors is then used to monitor the health and performance of the machinery. Even the slightest deviations from normal operating conditions can be identified promptly. As a result, we can anticipate potential failures before they occur, allowing for immediate preventive action and minimizing the risk of downtimes. Sensors are the eyes and ears of the predictive maintenance system, ensuring timely intervention and the smooth operation of equipment.
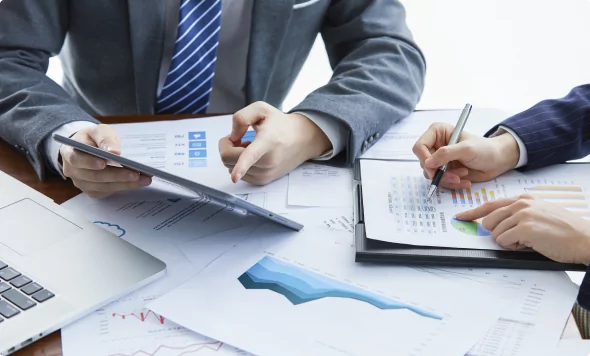
Second, there is historical data
AI algorithms, like machine learning models, are trained using historical data from past equipment performance and maintenance records. These algorithms learn patterns, correlations, and trends to predict similar patterns in real-time data. The algorithms adjust and improve their predictive capabilities as more information becomes available.
Third, there are AI algorithms
And here comes the star of the show: artificial intelligence in manufacturing. Analyzing sensor and historical data, AI algorithms can identify how machines are operating and not only detect failures but also predict potential malfunctions in the future. This proactive approach allows for prior intervention instead of acting when errors occur.
The beauty of AI algorithms lies in their continuous learning. With every new downtime case, they gain valuable insights, refining their predictions and becoming even more accurate over time.
Moreover, these AI models can be customized to meet specific needs and seamlessly integrated with other applications, creating advanced AI-based predictive maintenance systems tailored to your factory’s requirements.
The gains of predictive maintenance
Here are some reasons why predictive maintenance is gaining popularity in manufacturing:
- Cost savings: Predictive maintenance minimizes the likelihood of costly unplanned production line failures and downtimes and contributes to a longer machinery lifespan.
- Improved safety and quality: By addressing potential issues proactively, manufacturers can maintain a safer and more reliable production environment.
- Better asset management: With data on equipment health, manufacturers can optimize equipment lifespans, plan for replacements, and allocate resources more effectively, for example, by keeping an appropriate level of spare parts.
- Environmental benefits: By optimizing equipment performance, predictive maintenance can help reduce energy consumption and, thus, the factory’s environmental impact.
Becoming a smart factory
Predictive maintenance is crucial in the journey toward achieving a fully smart factory. It falls under the umbrella of the Industry 4.0 approach, which represents a significant departure from traditional manufacturing methods towards more interconnected, intelligent, and data-driven processes. In smart manufacturing setups, machines, devices, and sensors are connected and can communicate with each other through the IoT.
Despite its high-tech nature, predictive maintenance is widely used in manufacturing industries worldwide. As technology and data analytics continue to advance, an increasing number of manufacturing companies are adopting predictive maintenance as a pivotal part of their ongoing strategies.