AI technical analysis isn’t just a checkbox; it’s an essential step in forging effective AI solutions. Just like dissecting a high-performance engine, it involves meticulously examining the system’s inner workings: architecture, algorithms, models, and underlying technologies. This deep dive serves a critical purpose: uncovering the AI solution’s shining strengths and hidden weaknesses. More importantly, it proactively identifies potential threats and risks that could surface during implementation, mitigating their impact before they derail your project.
Don’t trust AI blindly
In AI we trust, but should we really? Artificial intelligence has quietly slipped into our daily lives, weaving itself into our routines and assisting us in ways we might not even recognize. From personalized news feeds to smart assistants, AI technology has become an invisible thread in the fabric of our everyday lives.
However, despite its growing presence, there’s a risk of mistaking its capabilities for sentience. It’s crucial to remember that AI, no matter how advanced, remains a tool designed by humans. That is why technical analysis in AI is so vital.
AI Technical Analysis: Understanding its benefits and limitations
Technical analysis in AI is crucial for:
- Building dependable and trustworthy AI systems
- Extracting valuable insights and predictions from complex data
- Making informed decisions across various domains
- Boosting innovation and efficiency in multiple fields.
However, it’s essential to acknowledge AI technical analysis’ limitations:
- Data quality and bias: AI models are only as good as the data they’re trained on. Biases within data can lead to biased outputs, highlighting the need for responsible data collection and analysis.
- Interpretability: Understanding how complex AI models arrive at their predictions can be challenging, which raises concerns around explainability and trust in AI decision-making, which we mentioned in the previous paragraph.
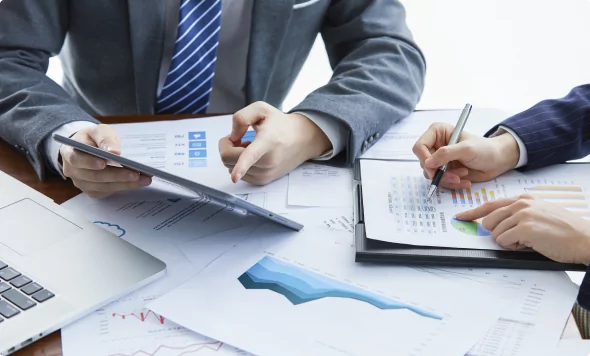
The components of AI system analysis
AI technical analysis refers to evaluating the inner workings of an AI system. It involves dissecting its:
- Architecture: How is the system designed and constructed?
- Algorithms: What logic and methods does it use?
- Models: What data representations and learning models are employed?
- Technologies: What tools and frameworks are utilized?
The goal is to assess the AI system’s:
- Strengths: What does it do well?
- Weaknesses: Are there any limitations or vulnerabilities?
- Suitability: Is it fit for its intended purpose?
- Security: Does it adequately protect data and privacy?
This type of analysis helps identify bottlenecks, performance issues, and security risks for proactive mitigation. It also allows developers to optimize the system, enhancing its capabilities and performance, which is crucial for developing and applying AI effectively.
AI technical analysis vs. AI due diligence
Both AI Technical Due Diligence and Technical Analysis in AI deal with evaluating artificial intelligence systems, but they have different aims, approaches, and outcomes. Understanding the differences between them is crucial for assessing AI systems effectively. Below, you will find a comparison:
AI Technical Analysis
- Purpose: AI Technical Analysis aims to assess and improve artificial intelligence models, algorithms, and datasets to achieve specific objectives or outcomes.
- Scope: It analyzes the system’s algorithms, models, and data used for predictions or decision-making.
- Stakeholders: It is used by developers, researchers, and AI system users to improve its performance and optimize its outputs.
- Outcome: It helps identify factors impacting the AI system’s effectiveness, allowing for adjustments and improvements.
AI Due Diligence
- Purpose: The main goal of the AI Technical Due Diligence is to uncover the AI solution’s strengths and weaknesses and identify any potential risks that could arise during its implementation. It provides insights into the feasibility and long-term viability of the AI solution.
- Scope: It evaluates an AI system’s feasibility, reliability, and potential. It involves a comprehensive assessment of AI’s concept and technical aspects, including tech stack, architecture, algorithms, datasets, model performance, and legal and regulatory compliance.
- Stakeholders: Typically used by investors, acquirers, or businesses considering adopting an AI solution.
- Outcome: Provides a comprehensive assessment of the AI system’s strengths, weaknesses, and suitability for the intended purpose
Read more about the key elements of technical analysis in AI due diligence.
Is AI Technical Analysis the “X-Ray” vision businesses need?
While the term “AI Technical Analysis” might not be universally used, the underlying concepts and processes are becoming increasingly important for businesses building AI-powered products. The way they use these approaches depends on their specific needs and context.
We observe that evaluating AI solutions in the current business realm is more common for external investment/acquisition rather than internal development. Investors are more aware of the need to assess the system’s strengths, weaknesses, and potential risks before committing resources. In the case of developing their own AI systems, businesses might rely more on internal quality assurance and testing processes alongside ongoing monitoring.
As AI becomes more complex and integrated into businesses, we can expect Technical Analysis to become even more widespread. Ultimately, technical analysis in AI is a powerful tool, but using it responsibly and ethically is vital, considering its limitations and potential biases.