As a manufacturing leader, you constantly seek innovative ways to enhance efficiency, reduce costs, and maintain a competitive edge. The advent of Generative AI (GenAI) offers transformative potential, and insights from Gartner and EY can help you navigate this complex landscape to drive substantial ROI while managing associated risks. This blog post delves into the value of generative AI in manufacturing, highlighting key use cases, addressing feasibility and risk management, and presenting a roadmap for successful implementation.
The Promise of Generative AI in Manufacturing
Generative AI represents a significant leap forward in technology, akin to the impact of the internet on research and inquiry. Gartner’s Report emphasizes that GenAI can enable profound business process changes in manufacturing by providing new, data-driven insights and recommendations. However, the adoption of GenAI must be approached with caution, ensuring thorough validation and verification of its recommendations.
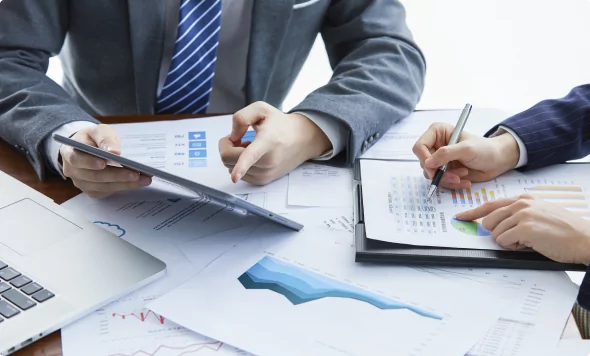
Understanding ROI and Risks
Implementing GenAI in manufacturing promises several benefits:
- Increased Revenue: By identifying new revenue opportunities and enhancing existing ones.
- Improved Efficiency: Achieving or exceeding performance goals with fewer resources.
- Managed Risk: Reducing uncertainties and improving agility to respond to market disruptions.
- Nonfinancial Value: Supporting goals like innovation, sustainability, and community development.
However, there are risks to consider:
- Technical Feasibility: Assessing whether current infrastructure can support GenAI solutions.
- Internal Readiness: Ensuring organizational willingness and capability to implement and trust GenAI.
- External Readiness: Considering legal, regulatory, and public opinion factors.
Key GenAI Use Cases in Manufacturing
Based on Gartner’s Use-Case Prism and additional research, here are ten highly feasible GenAI use cases that can significantly impact manufacturing operations:
1. Data Insights
Large language models (LLMs) can analyze vast amounts of data to uncover new revenue opportunities. By examining internal and external data, LLMs can connect the dots between company capabilities, market trends, and customer needs. This allows for better strategic decision-making and targeted marketing efforts.
ROI Potential: Increased revenue through better understanding of customer behaviors and market dynamics.
Risks: Data privacy concerns, potential misinterpretation of data leading to incorrect decisions, and reliance on data quality.
2. Robots for Hazardous Conditions
GenAI enables robots to autonomously inspect and address safety hazards in environments dangerous for humans. This improves operational efficiency and workforce safety.
ROI Potential: Enhanced employee safety and reduced operational costs.
Risks: High implementation costs, potential technical failures in hazardous conditions, and regulatory compliance challenges.
3. Materials Processing Efficiency
Integrating IoT sensors with GenAI can optimize materials processing by monitoring factors like vibration, temperature, and humidity. This leads to efficiency gains, reduced waste, and enhanced production quality.
ROI Potential: Faster production, improved equipment performance, and reduced waste.
Risks: High initial implementation costs, dependency on sensor accuracy, and potential technical failures.
Read also: When Generative AI Isn’t The Right Choice For Your Company?
4. Curated Shopping and Delivery
Connecting ChatGPT plugins to in-house AI technology can provide an AI-powered shopping experience, akin to a personal shopper. This enhances customer satisfaction and retention by personalizing the shopping experience.
ROI Potential: Improved customer satisfaction and retention through personalization.
Risks: Privacy issues related to customer data, potential inaccuracies in recommendations, and customer trust concerns.
5. Mechanical Design Optimization
GenAI can accelerate component design processes by optimizing for weight, cost, and performance. This reduces development time and costs while improving product quality.
ROI Potential: Faster product delivery and increased revenue.
Risks: Inconsistent design results, potential over-reliance on AI for critical design decisions, and integration challenges with existing design processes.
6. Factory Asset Effectiveness
GenAI can analyze production data to detect inefficiencies and anomalies, ensuring maximum utilization of equipment and resources. This improves overall production efficiency and quality.
ROI Potential: Increased efficiency and cost savings through anomaly detection.
Risks: Data integration challenges, potential false positives/negatives in anomaly detection, and over-dependence on AI for critical operational decisions.
7. Guided Machine Maintenance
GenAI can create user-friendly maintenance guides, enabling non-engineering staff to perform routine checks and repairs. This reduces downtime and enhances productivity.
ROI Potential: Reduced downtime and improved team productivity.
Risks: Accuracy of maintenance recommendations, risk of improper maintenance leading to equipment damage, and need for ongoing updates to maintenance guides.
8. Product Quality Intelligence
GenAI can analyze production data to diagnose issues and recommend improvements, enhancing product quality and sustainability. This leads to better decision-making and increased efficiency.
ROI Potential: Reduced waste and increased throughput.
Risks: Dependence on data quality, potential misdiagnosis of issues, and resistance to change from quality control teams.
9. Software Development
Leveraging GenAI for software development can expedite code creation and improvement. While there are risks associated with AI-generated code, the potential for faster iteration and deployment cycles is significant.
ROI Potential: Faster iteration and deployment cycles, optimizing efficiency.
Risks: Security vulnerabilities in AI-generated code, potential lack of accountability for errors, and challenges in integrating AI-generated code with existing systems.
A Roadmap for Implementing Generative AI in Manufacturing
EY’s research provides a comprehensive roadmap to help manufacturing leaders implement GenAI effectively. Here are the key steps:
1. Identify Strategic Objectives
Start by clearly defining your strategic objectives. Understand how GenAI aligns with your overall business goals and what specific outcomes you aim to achieve. Use frameworks like Michael Porter’s Five Forces to assess competitive dynamics and potential AI impacts.
2. Assess Organizational Fit
Evaluate the current role of technology in your organization and how AI might enhance or conflict with your competitive strategy. Consider whether to integrate established AI solutions via APIs or develop bespoke models tailored to your specific needs.
3. Develop the Implementation Sequence
Prioritize AI initiatives using a modified Boston Consulting Group’s Priority Matrix, assessing each initiative’s impact, urgency, and complexity. Focus on high-impact, high-urgency, and low-complexity projects for quick wins.
4. Prepare the Organization for Change
Create a detailed roadmap that communicates the shared vision, key milestones, and timeline for AI adoption. Address data management, regulatory compliance, and cultural readiness to ensure smooth implementation. Focus on data availability, privacy, and security, while preparing your team for changes in their roles and workflows.
Generative AI in Manufacturing: A Conclusion
Generative AI offers a myriad of opportunities for manufacturing leaders to drive ROI and operational efficiency. By carefully considering the feasibility and risks associated with each use case, you can strategically implement GenAI to transform your manufacturing operations. If you’re ready to explore how GenAI can benefit your organization, consider scheduling a consultation with AI experts to tailor these solutions to your specific needs.
Embracing generative AI in manufacturing is not just about adopting new technology; it’s about transforming your approach to business, staying ahead of the competition, and driving meaningful results. With the right strategy and careful implementation, the potential benefits far outweigh the risks, positioning your organization for a future of innovation and growth. Contact NeuroSYS today and book a free 1-hour consultation with an AI expert to discover how generative AI can elevate your manufacturing operations.