Generative AI represents a transformative leap in the realm of artificial intelligence, with its ability to generate text, images, and other media that closely resemble human creation. The pace at which generative AI is being adopted across industries is nothing short of remarkable, driven by its advanced cognitive capabilities and context-aware outputs. However, this rapid adoption is not without its challenges, particularly concerning quality assurance, ethical considerations, and operational integrity. This article explores the critical role that Quality Engineering (QE) plays in ensuring the successful and safe adoption of generative AI within enterprise ecosystems.
The Rise of Generative AI in Enterprise Ecosystems
Generative AI is not just an incremental upgrade to existing AI technologies; it is a disruptive force with the potential to revolutionize both internal operations and customer-facing processes across industries. From AI-powered chatbots that enhance customer support to automated content generation that streamlines marketing efforts, the applications of generative AI are vast and varied. Enterprises are leveraging these capabilities to drive efficiency, innovation, and growth, often with the promise of significant returns on investment.
However, the inherent risk of improper adoption comes alongside the allure of generative AI. If not managed correctly, enterprises face potential pitfalls such as data breaches, biased outputs, and regulatory violations. The passive consumption of generative AI – where enterprises indirectly utilize AI through existing technology platforms without active investment – further complicates the landscape. This unregulated adoption can lead to unintended consequences, including data leakage and security vulnerabilities, underscoring the need for stringent quality controls.
Read also: When Generative AI Isn’t The Right Choice For Your Company?
The Role of Quality Engineering in Generative AI
Quality Engineering (QE) has long been the backbone of ensuring that technology systems perform as intended, free from defects and vulnerabilities. In the context of generative AI, the role of Quality Engineering is even more critical. As enterprises integrate generative AI into their workflows, the quality function must evolve to address the unique challenges posed by this technology.
1. Ensuring Digital Safety and Security With Quality Engineering
One of the primary responsibilities of QE in the realm of generative AI is to strengthen the quality processes that safeguard against digital security risks. This involves expanding the scope of the quality function to conduct thorough evaluations of generative AI systems, ensuring that they are robust, secure, and compliant with regulatory standards. For example:
- enterprises must validate that generative AI tools do not inadvertently expose sensitive data or violate intellectual property rights,
- moreover, the quality function must implement continuous monitoring protocols to detect and mitigate any emerging risks associated with generative AI use.
2. Aligning Quality Functions with Generative AI Objectives
The strategic alignment of the quality function with generative AI objectives is crucial for realizing the full potential of this technology. QE teams must work closely with enterprise stakeholders to identify high-impact use cases for generative AI and assess their feasibility from a quality perspective. This includes evaluating the adaptability of existing workflows to accommodate generative AI and ensuring that the data used to train AI models is of the highest quality.
Generative AI can also enhance the productivity of QE processes by automating routine tasks and optimizing test strategies. For instance, generative AI can autonomously generate test cases based on contextual understanding, reducing the time and effort required for manual testing. This synergy between generative AI and QE not only accelerates the development cycle but also improves the overall quality of the end product.
Read also: From PoC to Production: Overcoming Challenges in AI Projects in Practice
3. Addressing Ethical and Regulatory Concerns
The ethical implications of generative AI are a growing concern, particularly in terms of bias, fairness, and transparency. QE must take a proactive approach to address these concerns by implementing governance frameworks that ensure ethical AI use. This includes developing guidelines for responsible AI deployment, such as preventing discriminatory outcomes and ensuring transparency in algorithmic decision-making.
Furthermore, enterprises must navigate a complex regulatory landscape as they adopt generative AI. Different regions have varying regulations concerning data privacy, intellectual property, and AI use, making it imperative for QE to stay abreast of these evolving standards. By aligning with regulatory requirements, QE can help enterprises avoid costly legal repercussions and maintain stakeholder trust.
4. Future-Proofing Generative AI Adoption
As generative AI continues to evolve, so too must the quality function. Enterprises must invest in developing new roles within the QE function that are specifically tailored to the needs of generative AI. For example, roles such as Generative AI Quality Engineers, Data Scientists in Test, and Explainability Specialists will become increasingly important as enterprises seek to ensure the reliability and transparency of AI-generated outputs.
Moreover, enterprises must consider the sustainability of their generative AI initiatives. The computational power required to train and deploy large AI models has a significant environmental impact, and QE must play a role in mitigating this by promoting the use of right-sized models and avoiding unnecessary overtraining. By adopting a proactive approach to IT sustainability, QE can help enterprises balance the benefits of generative AI with the need to minimize their carbon footprint.
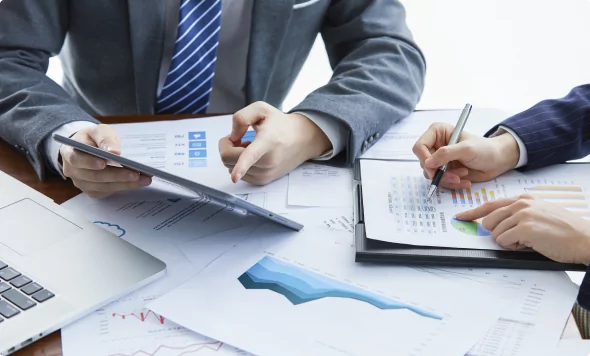
Generative AI Success with Quality Engineering: A Conclusion
The successful adoption of generative AI hinges on the effective integration of quality engineering throughout the AI lifecycle. From ensuring digital safety and aligning AI objectives with quality standards to addressing ethical concerns and future-proofing adoption strategies, the role of QE is indispensable. Enterprises that embrace a quality-first approach to generative AI will not only safeguard their operations but also unlock the full potential of this transformative technology, setting themselves apart in an increasingly competitive landscape.
As generative AI becomes more entrenched in enterprise operations, the need for rigorous quality assurance will only grow. By leveraging the strategic insights and interventions of the quality function, forward-thinking enterprises can maximize the benefits of generative AI while mitigating its risks, ensuring a sustainable and successful AI-driven future.
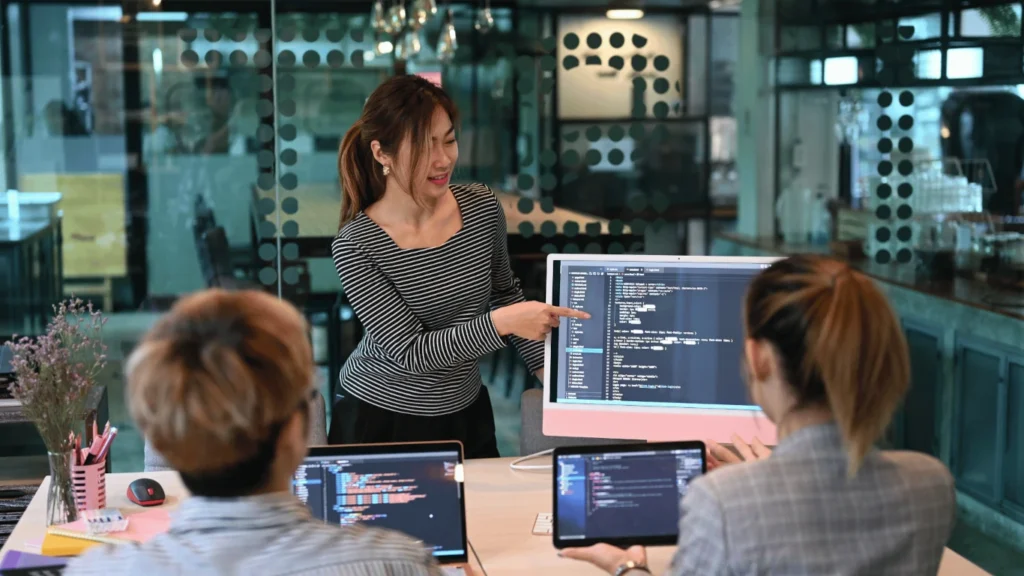
Explore the Potential of Quality Engineering with Free Expert Consultations
Unlock the transformative capabilities of generative AI for your business. NeuroSYS offers complimentary consultations with our AI specialists to help you discover tailored solutions that drive innovation, streamline operations, and enhance business performance.
Take this opportunity to gain valuable insights and explore how AI can strategically impact your organization.
Book your 1h free consultation today to start your journey towards AI-powered growth.