Downtime is one of the industry’s biggest challenges, generating millions of losses for enterprises from various sector branches. Various studies emphasize that the costs incurred due to unplanned downtime run into billions of dollars. For example, each hour of machine “rest” costs the manufacturing and automotive industries as much as $260,000 per hour (source). Therefore, the widespread introduction of technological achievements, such as predictive maintenance and AI, especially machine learning, is a must-have of Industry 4.0. In this guide, we will focus on implementing predictive maintenance using machine learning.
Predictive Maintenance (PdM) – How does it work?
Predictive maintenance (PdM) uses intelligent algorithms to forecast the remaining lifespan of machinery and components. By integrating various data sources with real-time hardware analysis, PdM enables companies to shift from scheduled maintenance to usage-based approaches. Thanks to predictive maintenance using machine learning, the service schedule depends on the actual use and condition of the machines.
For instance, in manufacturing, PdM can predict equipment failures by analyzing vibration and temperature sensor data, allowing proactive intervention to prevent costly downtime. Similarly, in the aviation industry, PdM can forecast engine failures based on historical performance data, enabling airlines to schedule maintenance during non-operational hours minimizing disruptions to flight schedules. These examples illustrate how PdM, an example of AI in manufacturing, as well as data analytics, revolutionizes maintenance practices across diverse industries, optimizing asset performance and reducing operational costs.
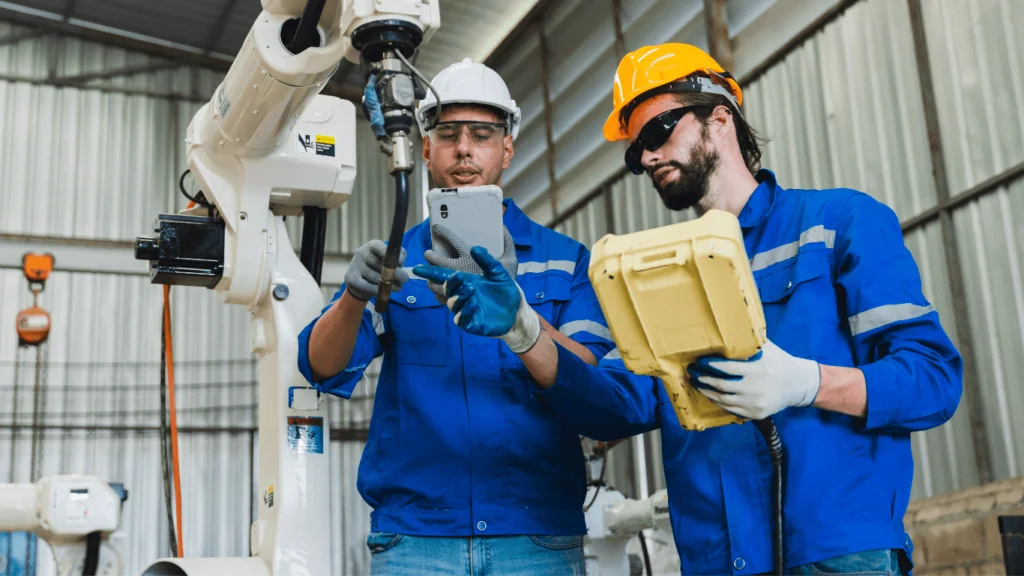
Predictive Maintenance vs. Preventive Maintenance
The concept of predictive maintenance was born out of the shortcomings of traditional preventive maintenance, which often fell short. The problem with fixed maintenance schedules is their inflexibility, leading to excessive maintenance that, instead of reducing downtime, actually prolongs it. Despite efforts to avoid the most intense periods, predicting them in advance is not always feasible. This results in manufacturers grappling with machine downtime during peak production periods. However, with the advent of predictive maintenance, there’s hope for a more efficient and effective approach.
The shortcomings of maintenance based on fixed schedules are evident. Research by the ARC Advisory Group has revealed that up to half of maintenance funds allocated to predetermined schedules are wasted. Furthermore, only 18% of equipment failures are directly linked to age or use, with 82% being random. This challenges the conventional belief that more frequent inspections lead to less downtime.
Amidst these challenges, visionary industrial entrepreneurs have stepped up, advocating for a more progressive approach to maintenance management. Their foresight and innovation have given birth to predictive maintenance, a game-changing solution that promises to revolutionize our industry
How Is Predictive Maintenance Using Machine Learning?
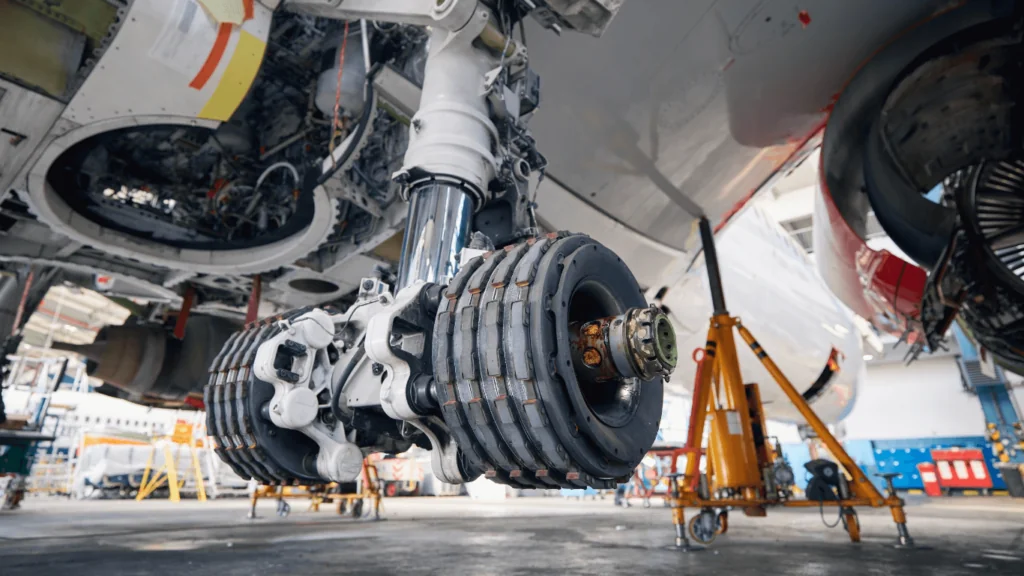
In short, predictive maintenance (PdM) uses intelligent algorithms, artificial intelligence, and data analytics to predict the remaining life of machines and their components. PdM combines multiple data sources with analysis and uses real-time or near-real-time hardware. PdM uses built-in intelligent sensors (e.g., vibration, temperature, and electric current sensors) and a combination of calculations in local devices with cloud computing to detect potential problems. Local or remote software based on the readings constantly analyzes the condition of the machines.
Using advanced modeling technologies and machine learning software solutions, PdM takes asset monitoring to the next level. It analyzes hundreds of process parameters over time and compares them to historical resource data. Thus, predictive maintenance using machine learning provides operators with better information earlier, giving them more time to solve problems and avoid failures.
Benefits of Machine Learning in Maintenance Optimization
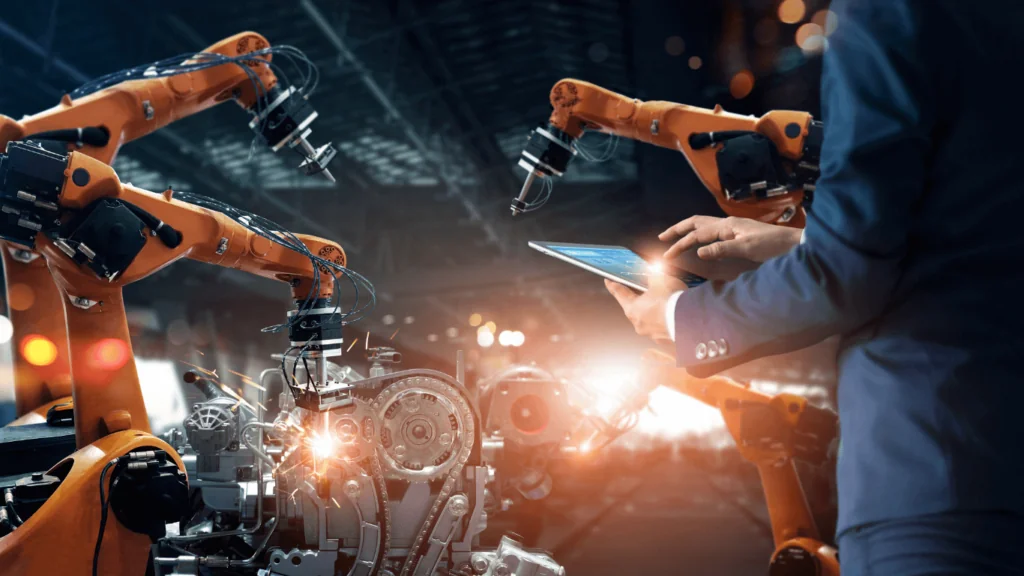
Predictive maintenance is gaining popularity mainly because it offers the opportunity to realize significant savings by performing manual inspections only when necessary. Predictive maintenance using machine learning also reduces the costs of taking machines out of use. Moreover, it offers sophisticated tools to forecast equipment failures and optimize maintenance schedules. Supervised learning algorithms, including regression and classification, analyze historical data to identify patterns indicative of impending failures, enabling proactive intervention. On the other hand, unsupervised learning algorithms, such as clustering and anomaly detection, sift through vast datasets to uncover irregularities or outliers, signaling potential issues.
In addition, reinforcement learning introduces dynamic maintenance scheduling by iteratively adjusting strategies based on real-time feedback, ensuring optimal resource allocation. By harnessing these diverse algorithms, organizations can predict equipment failures precisely and streamline maintenance operations, minimizing downtime and maximizing efficiency.
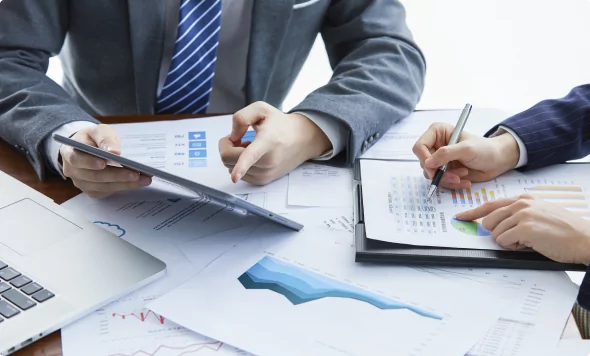
Implementing Predictive Maintenance Using Machine Learning
The goal of implementing predictive maintenance should always be KPIs such as operational efficiency, reducing downtime, and financial savings. To achieve this, it is worth preparing an implementation strategy in advance, the outline of which is presented below:
Data Acquisition and Preprocessing
Data acquisition and preprocessing are essential in predictive maintenance using machine learning as they ensure the quality, relevance, and consistency of the data used to train and deploy predictive models. By optimizing data quality and preparing it for analysis, organizations can maximize the effectiveness of their predictive maintenance initiatives, improving equipment reliability, reducing downtime, and lowering maintenance costs. Here are some guidelines for doing it correctly:
- Machine-vision predictive maintenance requires a systematic approach to data collection and pre-processing. To effectively collect this data, you must set up systems to collect real-time data from various production sources.
- The process begins by identifying the basic data types critical to predictive maintenance. These should include, among others, data from sensors, equipment logs, maintenance records, and environmental factors.
- Maintenance experts can leverage Internet-of-Things sensors, SCADA systems, augmented reality solutions, and other monitoring devices to facilitate end-to-end data capture.
- After collecting the data, it must be pre-processed. This filters out outliers and inconsistencies, standardizing data ranges by normalizing and extracting appropriate model features for analysis.
- Ensuring data quality and integrity throughout the process is also paramount. Implementing measures to ensure data accuracy, consistency, and completeness increases the reliability of predictive models, laying a solid foundation for effective maintenance strategies.
Model Training and Evaluation
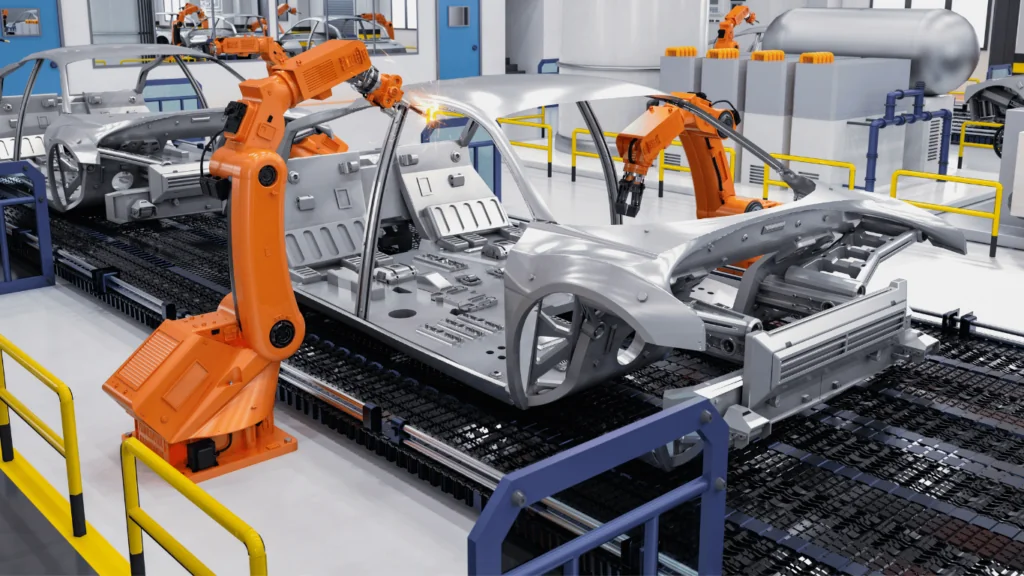
Maintenance managers oversee the implementation of predictive maintenance using machine learning, and practical model training and evaluation are paramount. Here are some tips on how to conduct training and model assessment:
- First, establish a robust framework for training machine learning models using preprocessed data. This involves selecting suitable algorithms tailored to predictive maintenance tasks, such as regression, classification, clustering, or reinforcement learning.
- Then, model evaluation and validation techniques will be used to ensure their effectiveness and reliability.
- Employing cross-validation methods and performance metrics such as accuracy, precision, recall, and F1-score can provide insight into model performance and efficacy.
- Align evaluation metrics with your KPIs, such as minimizing downtime, maximizing equipment lifespan, and optimizing maintenance costs.
- By prioritizing metrics that resonate with overarching objectives, manufacturing companies can gauge the success of predictive maintenance initiatives and make informed decisions to drive operational efficiency.
Implementation Challenges and Solutions
Before implementing PdM into daily operations, it’s crucial to identify and anticipate common hurdles, including imbalanced datasets, data drift, model interpretability, and scalability issues. To overcome these challenges, the maintenance experts can implement techniques such as oversampling or undersampling to address imbalanced datasets, while continuous monitoring of data drift ensures model accuracy over time.
Additionally, successfully implementing predictive maintenance using machine learning requires constant monitoring and updating of models. Real-time monitoring of equipment condition and performance allows for right-on-time interventions. This is also when new data helps update ML models, ensuring continuous optimization.
Making Maintenance Smarter: AI Workshops and Free Consultations
If you want to implement predictive maintenance using machine learning or other solutions enabling your enterprise’s digital transformation, use professional AI consulting or identify areas that provide the highest ROI thanks to artificial intelligence discovery workshops.
AI workshops offer a helpful starting point for companies looking to improve their maintenance practices. These workshops teach about using AI for predictive maintenance, which means fixing things before they break. You’ll learn how AI can fit into your company’s operations. You’ll understand how AI can save money and improve your work by seeing real examples. Plus, there’s a bonus: a free 1-hour consultation with NeuroSYS! In this type of session, experts will give you advice just for your company. They’ll help you figure out the best ways to use predictive maintenance.
To learn more about the AI workshop offer, download these 4 real-life case studies:
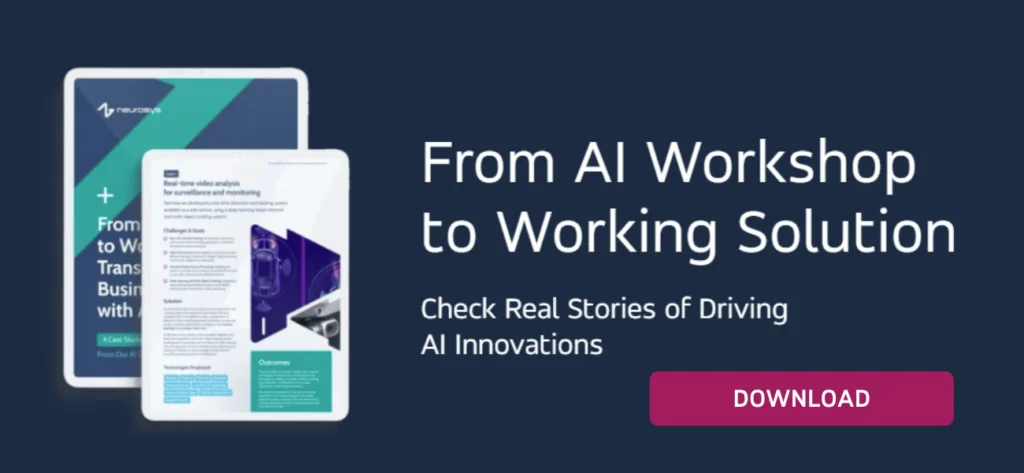
.