Interview with Tomasz Kowalczyk, CEO of NeuroSYS
In this interview, Tomasz Kowalczyk shares observations from audits carried out by NeuroSYS at multiple factories and gives real-life examples of issues and solutions provided by NeuroSYS.
NeuroSYS specializes in conducting technical audits to identify problems that reduce factory production efficiency and build modern IT solutions, including those using AI technology. Our experience and knowledge allow us to effectively support manufacturing industry leaders in optimizing processes, reducing downtime, and improving operational efficiency.
What are the most common technological problems that factories face?
Tomasz Kowalczyk: These problems are very different and depend on the type of production, the size of the company, the type of machines and many other factors. One common challenge is related to data integration. Note that factories focus mainly on collecting and processing data related to the basic production process. They want to achieve high-quality, safe production that complies with industry standards. However, they lack IT resources and analytical competencies for more advanced analysis of the collected data and their integration. This makes it difficult to make better business decisions, especially optimizing production processes, downtime, hardware problems and failure prediction.
Many factories use Process Control Systems (PCS) to monitor production parameters such as pressure, temperature and number of equipment cycles. In turn, Computerized Maintenance Management Systems (CMMS) manage equipment maintenance and repairs information. The problem arises when these systems are not integrated with each other. For example, in one of our projects, the factory had a complete history of pump cycles in the PCS system, but information about the replacement of this pump was recorded in the CMMS. When a pump failed, the PCS system had no information about the pump replacement, leading to incorrect data and making it difficult to make good maintenance decisions.
What are the main challenges associated with integrating Process Control Systems and Computerized Maintenance Management Systems? Is this a common problem in manufacturing companies?
Process Control Systems (PCS) and Computerized Maintenance Management Systems (CMMS) are two types of basic systems operating in manufacturing companies. However, they are often not integrated with each other.
But that’s not the only problem. During our audits, we also saw factories where there is no CMMS and the maintenance management is done entirely on paper. Some factories do not even have PCS. This depends heavily on the company’s scale and the industry in which it operates. In the chemical or pharmaceutical industries, production lines have sensors installed at many stages of production because monitoring indicators such as pressure or temperature affect the fulfilment of restrictive standards for admitting products to market circulation. Here, PCS class systems are a norm.
However, the situation is different in more mechanical production, such as in the automotive industry. Some smaller factories still perform most processes manually without an advanced production monitoring and automation system.
The main problems for the factories we cooperated with were the digitization of the process and the ability to monitor production throughout its entire length, i.e., all key parameters at individual stages. Even if the above-mentioned systems are implemented, the problem is their fragmentation and lack of integration.
Let me give you an example of one of the factories we audited. The PCS system was running locally, while the CMMS was global and in the cloud. This caused additional difficulties in communicating and integrating these systems. It also made it difficult to fully use the collected data to optimize processes and make business decisions.
Is the challenge of having factories operating under one brand and having a variety of systems and production lines common to large companies with multiple locations?
This problem particularly affects large companies with multiple locations. Factories usually have some decision-making autonomy, which leads to situations where different units have different production lines from different manufacturers and use different systems. However, these systems are generally not interconnected, further complicating the situation. One factory may use a CMMS, while other factories from the same company use completely different systems of the same class. The lack of consistency at a global level means that each factory operates a separate management system, making it difficult to coordinate and optimize processes centrally.
What technologies can help solve data integration challenges?
When integrating data in manufacturing, the solution may be advanced SCADA (Supervisory Control and Data Acquisition) systems, which enable data collection from various sources and their centralization. These systems can integrate data from PLC (Programmable Logic Controller), HMI (Human-Machine Interface) and other devices on the production line. Additionally, using technologies such as the Internet of Things (IoT) and data analysis using machine learning can help identify trends and predict failures.
An example is a factory where we helped install sensors on the production line to measure the number of containers produced and downtime. This data was then analyzed in real time, allowing for quick problem responses and process optimization.
From what we’ve seen, knowledge management is also a big issue that costs companies a lot of money. What should be done to fix this problem?
McKinsey research shows that the average maintenance engineer wastes about 30% of their work time searching for information on machine operation, part replacement, service procedures and standards. These studies confirm that engineers spend too much of their time finding appropriate documents and instructions. This problem is especially visible when an experienced employee leaves the company, leaving behind many unorganized documents.
An example we encountered was a situation in which an employee, after 30 years of working in a factory, left thousands of e-mails, documents and files on a shared drive. The new person taking over his duties had no idea how to navigate it.
We proposed a solution based on LLM (Large Language Models), which facilitated access to information and allowed searching all data collected on platforms such as OneDrive or Google Drive. LLM technology will enable you to quickly find mentions of specific projects in many documents, significantly reducing the time needed to search for information.
LLMs can also be used to verify the validity of procedures by automatically changing regulations. One of the chemical factories we cooperated with had thousands of procedures to comply with current regulations. Manual verification was time-consuming and error-prone. Here, our use of LLM allowed us to check the compliance of procedures with current regulations automatically. This, in turn, increased efficiency and reduced the risk of non-compliance with legal regulations.
I will also give another example of how using LLM technology for information management enables better use of collected data to optimize production processes. At one client site, retooling the production line took 5 hours due to poorly trained staff. After implementing appropriate training and process standardization, we shortened this time to 2 hours, significantly reducing downtime and improving efficiency.
It is worth adding that LMS class systems can be implemented locally – on company servers. They do not have to be a cloud solution, and a common concern that we encounter with our clients is processing confidential data in the cloud.
How does the digitalization of production processes affect production efficiency? What about the key KPI for manufacturing leaders such as OEE?
OEE (Overall Equipment Effectiveness) is a key parameter for manufacturing leaders that measures efficiency and resource utilization. This is a standard indicator that takes into account time, efficiency and the number of defective products. Optimal OEE should be close to 100%, which means that equipment and resources are being used to their maximum potential.
We have noticed that calculating OEE is still problematic in many factories, negatively affecting production efficiency. In one of the factories we audited, OEE was calculated in a way that lowered the comparison base. Instead of calculating productivity based on maximum production capacity 24/7, they relied on a production plan that included only two shifts.
In this factory, the OEE target was just over 40% of full production line capacity, which was a very low baseline. This method of calculating OEE allowed for artificial improvement of results, which, however, does not reflect real performance.
When we analyzed the data from this factory, we found that the actual efficiency was much lower. The problem was that there were no unified rules and processes for calculating production KPIs and precisely monitoring them.
Another example we encountered was a factory producing plastic elements. Here, operators had to manually record the number of items produced, which led to inaccuracies and errors. Managers often only found out about shortages at the warehouse level, which resulted in the need to organize additional shifts and work overtime to make up for the backlog. Such incorrect OEE calculations and the lack of precise data led to increased costs and problems with production planning.
What does declaration-based production actually mean and how have we helped customers improve it?
Declaration-based production means that operators manually report the results of their work. In practice, the number of items produced is recorded by operators on paper or similar. We have seen such a system in various factories, including one in Poland and one in Norway. The data is often unreliable, making it difficult to make production decisions based on it. Operators often report data that is consistent with the production plan, but does not necessarily correspond to reality. Managers know this data is unreliable, but they do not have the tools to verify it.
One of the solutions from our portfolio was implementing a packaging line monitoring system in one of the factories. We have installed sensors that measure the number of containers and packages passing through the production line in real time. This data is automatically saved and reported. Additionally, our system sends SMS notifications to the maintenance department and managers when a machine stops working. Previously, such downtimes were reported manually on the board, which was imprecise and made data analysis difficult. This client now has accurate and up-to-date information on production status and its technicians can respond to issues faster, significantly improving production efficiency.
What are the main gaps in the digitalization of production processes and where should digitalization be accelerated to see positive changes as quickly as possible?
These deficiencies depend largely on the type of production. If production is highly manual and lacks dedicated automation machines, there are two main ways to improve it.
The first is automating the process by designing a new production line that automatically performs most tasks. Every new production line should integrate sensors that measure the amount of product input, output, and waste then monitor whether the machine is working properly. The key element here is microcontrollers (PLC), which manage the entire machine and collect data that can be sent to external SCADA systems.
The second option is adding sensors to an existing production line and transmitting data to PLC systems. These systems collect data in real time, managing the process with cycle times of up to 100 milliseconds. Data from PLC systems can be sent to a computer, and SCADA systems collect this data, enabling statistical analysis of many machines. Accelerating digitalization in such cases brings immediate benefits in the form of better monitoring, waste reduction, and machine uptime optimization.
Thanks to automation and the use of PLC and SCADA systems, factories can identify problems faster, take appropriate corrective actions and significantly improve production efficiency. This approach delivers quick and measurable results, which is crucial for manufacturing leaders looking to increase efficiency and reduce operating costs.
Could you give an example of a specific improvement that has provided significant benefits to our customers?
In one of the audited factories, the biggest production bottleneck was the Powder Transfer System (PTS). The PTS is a machine resembling a large vacuum cleaner that pulls powder from buckets into the process line where it is mixed with other reagents. Although this mechanism is quite simple, the machine often broke down, resulting in many hours of downtime and the need to work overtime to make up for lost production.
Our audit showed that the membrane in the PTS, responsible for creating negative and overpressure, was often clogged or damaged. We have implemented a pressure monitoring system on both sides of the membrane. Thanks to this, we were able to predict failure before actual damage occurred, which allowed for faster and less expensive component replacement. We reduced the downtime from 5-6 hours to just 1 hour, significantly improving production efficiency. Our experience shows that factories, even those with modern production lines, often still struggle with efficiency problems. So where is the problem?
This project is a typical example of predictive maintenance (PdM), where monitoring key parameters allows components to be replaced before they fail, which can result in significant time and cost savings.
Our experience shows that often factories – even those with modern production lines – still struggle with efficiency problems. So where does the problem lie?
During audits, we often discover that the so-called bottlenecks may result from an inappropriate way of “sensing” the production line and collecting data concerning analytical needs.
Here’s a real-life example. One factory had modern production lines equipped with sensors and PLC systems, as well as computers with HMI to monitor machine conditions. The packaging line had sensors counting the number of containers, but the data was unreliable. The sensor counted the containers before the quality control stage of stickers, which had to be glued to the container in a strictly defined way. The read data did not include waste resulting from incorrectly applied labels. As a result, the counter reported the production of e.g. 5,000 containers, while 1,000 of them could have been rejected as waste. The customer was aware of this problem but did not have the proper tools to fix it.
Our job was to thoroughly analyze this problem from a technological perspective and propose a solution. We added additional sensors to the packaging line and integrated them with the central system, continuously monitoring the production status, including quality control stages. As a result, the customer can now rely on accurate data, allowing for better management of the production process and waste reduction.
What are the key issues that leaders of manufacturing companies pay attention to?
This is primarily overtime work, waste reduction and downtime reduction. These are fundamental elements affecting production efficiency.
Let’s start with downtime. During the audit, the NeuroSYS team examines how often downtime occurs and what its causes are. It doesn’t always have to be a failure. Downtime is often caused by the need to retool production lines. In the case of lines that need to change their packaging (e.g. from 10 ml to 100 ml or half-liter bottles), downtime may last many hours. We then check whether the staff is properly trained and whether the retooling process is standardized. In well-managed factories, changeovers can take much less time, minimizing downtime.
Working overtime is the second problem. We ask the client how often overtime work occurs and what are its reasons. We analyze whether overtime results from insufficient efficiency of production processes or, for example, unplanned downtime.
When it comes to waste reduction, we analyze production processes in terms of the amount of waste generated. We check whether there are ways to reduce them, which directly impacts production costs and operational efficiency. We also evaluate the technological solutions used, such as production line monitoring systems, and analyze the scope and type of data collected.
How does NeuroSYS Technical Audit work and how can it help factories in their production optimization process?
The NeuroSYS technical audit identifies problems and proposes solutions to improve production processes. This is a process that begins with a thorough understanding of the client’s problems through on-site conversations and observations. Our team of experts analyzes production lines, identifies key challenges and suggests possible improvements.
We start the audit by walking around the factory and familiarizing ourselves with each stage of the production process. We devote the first day to identifying key challenges and problems, both technical and organizational. Then, based on the information collected, we analyze it and suggest possible improvements.
The next stage is a brainstorming workshop, during which we discuss our proposals with the client and prioritize them in terms of ROI and effectiveness. As part of the audit, we can also design and implement pilot projects, such as IoT systems for monitoring downtime or advanced data analyses using machine learning.
During the audit, we develop a list of ideas for proof of concept (POC) projects and assess their ROI, usability, prioritization, and valuation. We then work with clients to define the project’s scope and ensure that proposed solutions align with their goals.\
For example, in one of our recent projects, we implemented an IoT system that collects data and reports machine downtime to the maintenance department. This project was implemented in about a month at one customer location and turned out to be so effective that managers from other factories asked if they could implement it at their own sites.
What are the biggest challenges associated with applying AI in factories, and how do manufacturing leaders deal with them?
AI technology is often perceived as an expensive investment. Manufacturing leaders are sometimes confused about how and where to start implementing AI in their factory to achieve the highest return on investment (ROI). They often lack AI specialists on their internal teams. This, in turn, makes it impossible to apply an effective “use case hunting” approach and methodology, i.e. identify the most reasonable AI applications in your factories.
What most often stops companies from implementing AI?
Many companies think the only way to use AI is to make major changes to the production line to monitor it and collect appropriate data properly. Such an investment seems risky and very expensive. We propose a solution that allows for a significant step towards technological advancement and the use of AI without huge investments in sensors on the production line itself. This is a “Camera as a Sensor” system, which allows using cameras instead of traditional sensors to collect data without interfering with the production machinery. This enables companies to implement AI more flexibly and cost-effectively.
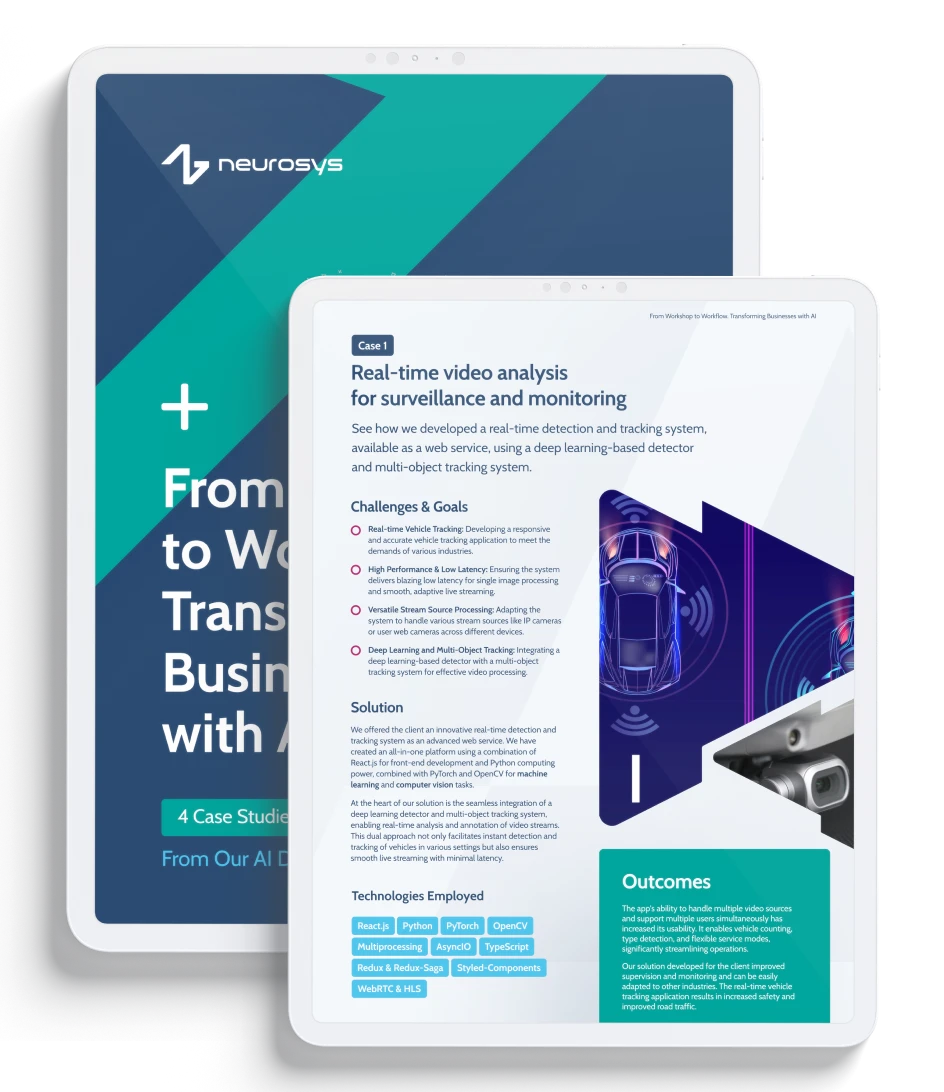