Interview with Marcel Kasprzak, Managing Director of NeuroSYS on data challenges in AI projects
NeuroSYS: Why is data so important in AI projects?
Marcel Kasprzak: Data is the foundation of every AI project. With the correct data, AI models can learn and function properly. Good data must be accurate, complete, up-to-date and well-labeled.
Recently, the approach to creating AI tools has changed significantly. In addition to the standard code-centric approach, where AI applications are built on principles similar to those of other software applications, we emphasize the data-centric AI approach more. This approach focuses on data to build better AI systems. AI-specific data management, synthetic data, and data labeling technologies play an important role here, aiming to solve many data-related challenges, including availability, volume, privacy, and security.
Can a lack of data completely block an AI project? Is it sometimes impossible to collect data?
Yes, a lack of data can be a serious problem. We experience this type of challenge in many of our implementations. For example, in a project for a pharmaceutical company asking for automated recognition of bacteria in Petri dishes, we did not have enough photos of infected samples. We had to prepare additional data, which was expensive and time-consuming but necessary for the model to work effectively.
Preparing additional data included inoculating bacteria, photographing samples, and marking bacterial colonies in these photos. As a result, from the initial 200 available photos, we expanded the database to 18 thousand pictures of Petri dishes. Then, to make processing easier, we cut out individual colonies, which resulted in almost half a million photos of the colonies themselves. This data was necessary to train the AI model effectively.
Another example is an automotive company that wanted to detect small scratches on luxury car parts. During the year, the company had very few defective elements, which did not allow for effective training of the AI model. In such cases, we sometimes have to accept the limitations of the technology or look for alternative solutions, for example, by generating artificial data.
Can artificial data replace real data in training AI models?
Artificial data can complement real data, but they must be created carefully. I will give an example from our research and development project, which proves how promising the process of preparing artificial data has for effective training of AI models.
In the project “Generation of microbial colonies dataset with deep learning style transfer”, the results of which were published in Nature.com, we combined traditional computer vision techniques with a deep learning transfer algorithm. Thanks to this, we created a microbial data generator using only 100 real images. This approach allowed us to generate synthetic data sets that can train state-of-the-art deep learning object detectors. In this project, careful preparation of synthetic data provided our model with high efficiency in detecting microorganisms. This scientifically confirms that synthetic data can improve the training process of AI models when real data is insufficient.
Additionally, the use of generative AI to create synthetic data is growing rapidly, which reduces the burden of acquiring real-world data so that machine learning models can be trained effectively. Gartner predicts that by the end of this year, 60% of AI data will be synthetic, which will help simulate reality and future scenarios and reduce the risks associated with AI. Artificial data can be helpful, but it cannot completely replace real-world data.
Read also: When Generative AI Isn’t the Right Choice for Your Business: An Expert’s Take on Gartner’s Insights
What are the challenges associated with data acquisition in practice?
Here, I will draw on the experience we gained in a project for a mining company. Our task was to develop a concept for a system that detects bearing failures on underground conveyor belts. The client’s initial idea, which involved using drones with thermal imaging cameras, failed due to difficult conditions. Therefore, we proposed an alternative solution in the form of small sound recording devices that could detect differences in noise indicating an impending failure and locate where a given anomaly occurs. This example shows alternative approaches to data acquisition, the source of which can be both vision and sound.
Another example of the challenge in obtaining appropriate training data can be our implementation for a shrimp production company. Here, the goal was to estimate the biomass, i.e. the number of shrimp in water tanks, as precisely as possible. In this project, we worked with a client who provided us with photos of tanks. We had to describe them appropriately so that the AI system could recognize shrimp in various situations, even when they were partially covered. This work involved carefully labeling each photo, indicating where the shrimp were and their condition. We also described possible obstacles and interferences in the data used to train the AI system, so that the tool could learn to recognize shrimp in realistic conditions. Another major challenge is the scatter and lack of data integration.
So how to deal with data being scattered across different systems?
Data scattering is a challenge we often encounter in our companies. An example is our predictive maintenance project for an industrial equipment manufacturer. In this project, data on machine cycles was in one system, and data on component replacements was in another. We could only build an effective predictive model that predicted potential production interruptions after combining this data. This work required integrating different data sources and creating a unified database, which allowed for the effective acquisition of training data to feed the AI tool.
What are the most common mistakes when collecting training data for an AI-based tool?
One of the most common mistakes is poor data selection, which does not ensure its diversity and only reflects some potential cases occurring in reality. A good example is the project of recognize wolves and German shepherds, which has been described many times in online publications. The algorithm was supposed to learn to distinguish between these two species of animals. Still, it indicated a wolf every time it detected snow in the background because it just so happened that all the images it was trained on showed wolves in the snow.
The problem stemmed from the images’ improper preparation and lack of focus on key animal features. This shows how important it is to prepare the data carefully and thoughtfully. Inaccurate labeling can lead to creating a tool that does not work properly and does not meet its objectives.
What practical steps should be taken to ensure high data quality?
Of course, data quality is crucial. To illustrate this, let’s use our cooperation with a micromobility company. The project aimed to build an AI tool that could predict the demand for scooters in different places and times of the day, allowing for optimizing their deployment in the city.
The first step was to ensure that GPS data and user logs were accurate, complete and synchronized. We conducted a thorough data analysis to identify and fix any anomalies. We checked whether the GPS data matched the time and location of the user logs to eliminate inconsistencies.
Next, we cleaned data, removing any erroneous, duplicate or incomplete entries. We used advanced automatic anomaly detection tools to help us continuously monitor and correct any anomalies in the data.
These efforts ensured that the AI tool was based on reliable and consistent data, significantly improving its ability to predict scooter demand. Each project begins with a thorough data analysis to identify and fix potential issues, which is key to ensuring high data quality.
Finally, let’s talk about security. How to ensure data security in AI projects?
Data security in AI projects is becoming an increasingly complex issue, especially as they scale. One of the main challenges is managing data risks. The growing popularity of generative AI tools such as Stable Diffusion and ChatGPT is tempting employees to use these technologies to increase productivity. Without proper management and training, this can lead to leaks of protected information. Implementing rigorous security protocols is essential to minimize these risks.
Another challenge is protecting against external threats. AI models can be vulnerable to attacks such as Trojans or data injection attempts, disrupting systems and leading to erroneous results. Introducing advanced defense mechanisms, such as anomaly monitoring and regular security audits, is crucial to ensuring data integrity and security.
Additionally, companies must comply with data protection regulations. As regulations become more stringent, organizations must ensure that their data management practices align with applicable regulations. This includes implementing appropriate access control mechanisms and data encryption and ensuring that all data processing is transparent and compliant with legal requirements.
In the context of AI, data security management requires an integrated approach that encompasses both technologies and organizational processes. It is important to build awareness and a culture of security among employees, train them regularly, and apply best practices in data protection.
Are You Facing Data Challenges in AI Projects?
If you are facing data challenges in AI projects, consider taking advantage of a 1-hour free consultation with an AI expert. During this session, you’ll gain valuable insights into how to tackle data-related issues, such as data scarcity, quality, and security. Our experts will provide practical advice tailored to your specific needs, helping you navigate the complexities of data management in AI. Whether you’re just starting out or dealing with advanced AI implementations, this consultation can offer the guidance you need to optimize your projects and achieve better outcomes.
Don’t miss this opportunity to successfully address data challenges in AI projects and enhance your AI strategies. Take advantage of professional support and book your free consultation today!
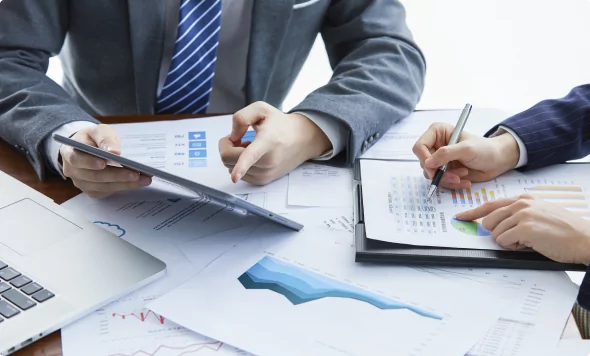