- Mistakes companies make with initial AI investments
- Identifying high-ROI AI applications
- Approaches and tools for successful AI projects
Interviewing Marcel Kasprzak, Managing Director NeuroSYS, on challenges in AI projects
In our conversation with Marcel Kasprzak, we explore the key factors that often hinder the success of AI projects. Apart from discussing challenges in AI projects, Marcel provides practical insights and hands-on tools to help companies overcome these challenges, avoid common pitfalls, optimize investment costs, and achieve desired business outcomes from AI initiatives.
NS: According to research, only 10% of AI projects in companies go from the testing phase to production. What is the reason for this?
Marcel Kasprzak: The main challenge is identifying projects with predictable costs and an accurate return on investment (ROI). Companies often cannot identify a project that would allow them to enter AI technology gradually. At NeurosSYS’s AI consulting, we recommend that clients implement smaller but well-defined projects that companies can improve and derive business benefits from, while learning how to manage organizational change. Engaging in extensive projects at the very beginning is risky in terms of knowledge, business processes, and employee readiness. The lack of a perspective of small, step-by-step implementations discourages companies from launching smaller but important AI initiatives that can be effectively scaled later.
Additionally, many companies lack AI implementation practices, which makes it difficult to identify specific use cases. The core of the issue is not recognizing AI models per such, but rather combining expertise with business process analysis and understanding the potential and limitations of data and equipment. Therefore, an important and recommended first step is AI Discovery workshops, which allow you to combine technical knowledge with a thorough understanding of the processes taking place in the company to properly design AI applications and estimate ROI.
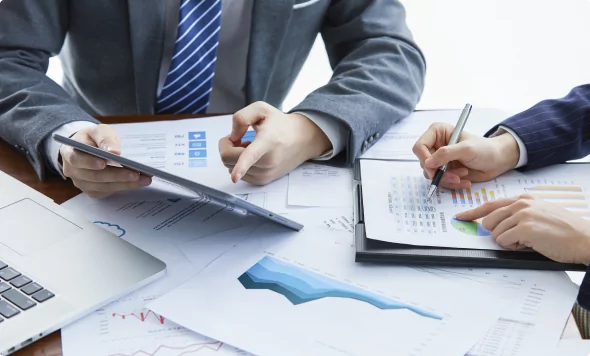
Can you give a specific example where the company had difficulty defining the use case and ROI?
An example is our cooperation with a pharmaceutical company that requested a solution to detect and count overturned vials on a conveyor belt that was transported to the filling machine. The company wanted to reduce production downtime and collect statistics on overturned vials. The previously installed sensors and vision systems were ineffective, and the company needed an additional AI-powered vision system. With our solution that detected and counted overturned packages, the company was able to better estimate the ROI, which allowed them to optimize the OEE (Overall Equipment Effectiveness) and increase production efficiency.
An additional example where the company had difficulty defining the use case and ROI was a project on recognizing and counting farmed shrimps. Our challenge was to accurately label and describe the vast amount of data presented in thousands of images. We focused on precisely labeling the tank images to ensure the model could recognize shrimp in various scenarios, even when they were partially covered. This example highlights the importance of thoroughly understanding the specifics of the business domain and properly preparing the data to train AI models.
Read also: AI Revolution In Aquaculture Technology
Apart from problems with understanding business processes in the context of AI technological capabilities, are there other factors that make it difficult to identify use cases and ROI?
Yes, and another key factor is effectively managing upfront costs and risks, as I will illustrate with the shrimp project mentioned above. A shrimp farm client was faced with challenges in accurately counting shrimp in tanks, which was necessary to estimate biomass to optimize feeding and, if necessary, administer medication during disease outbreaks.
During the AI Discovery Workshop, we recommended changes to the data collection phase of the PoC for this client. By replacing the expensive industrial camera with a smartphone, we significantly reduced the upfront costs. This strategy allowed the company to implement the PoC without a significant financial investment and to evaluate how AI could help with shrimp counting before committing to more advanced equipment. Our AI Workshop also helped the company gain a clearer understanding of the business benefits, including the quality and potential of the data that AI could bring to the case, and effectively estimate the return on investment, leading to more informed investment decisions.
Is there a universal performance indicator for AI projects? What factors influence it?
The basis for assessing the effectiveness of AI projects is ROI, and to accurately assess ROI, companies need to identify the key factors that drive its growth. When designing an AI algorithm, we first need to establish its operational parameters to achieve the target ROI. Only after this step can we move on to examining specific AI algorithm performance metrics. There is no universal KPI, as it varies depending on the specific use case. Furthermore, typical AI performance metrics such as MAE or MSE, which measure how closely predictions match actual results, are incompatible with traditional KPIs, making the problem much more difficult. Instead, effectiveness must be assessed through a lens that takes into account the unique requirements and goals of each business scenario.
To evaluate the effectiveness of AI projects, we can use the percentage of effectiveness as a primary indicator. However, its value is highly dependent on the specific business process conditions. For instance, a 90% effectiveness rate might be adequate for one application, while another may require 98% to meet its objectives. In some cases, a 97% effectiveness rate might seem impressive, but if the data processing takes 10 seconds and the production cycle demands 1-second responses, the solution becomes impractical.
Additionally, factors such as processing costs, energy consumption, and CO2 emissions play a crucial role in the overall assessment. Ultimately, the final solution must be justifiable not only in terms of effectiveness but also in terms of time, cost, and environmental impact. Sometimes it is necessary to reduce the effectiveness for faster processing, which on mobile devices can affect battery life and performance. Each case therefore requires an individual analysis to find a balance between effectiveness and other technical parameters.
Many AI testing projects stall due to low solution effectiveness. What causes this problem?
Perhaps the biggest reason for failure are AI data challenges such as lack of data to train AI models or its poor quality. Data is the foundation of every AI project – it allows AI developers to train models and determines how they will perform. It is very important to ensure that it is accurate, complete, up-to-date, and well-labeled.
Usually, AI data problems result from a lack of technical knowledge and insufficient understanding of the mathematics behind AI models. This affects the optimization of existing models, leading to their poor performance. AI is all about working with data, not just coding. Therefore, the key is to collect, process, and understand data, not just program the tool.
Other reasons for poor AI project performance include a shortage of application-specific customization and mathematical optimization. Neglecting these factors can lead companies to implement projects that are technically sound but have no real-world application. To truly improve work efficiency with AI, a deep understanding of business processes is essential.
Can you give an example of a PoC project that hit a dead end due to poor model performance, but was improved?
Yes, we had such a case. A pharmaceutical company wanted to count bacteria on Petri dishes using a vision system. Their previous solution was ineffective for three reasons. First, it relied on standard vision methods such as contrast and older AI models. Second, the system did not recognize cells on the edges of the dishes well. Third, it misidentified scratches as bacteria cells, causing many samples to be incorrectly flagged as contaminated.
When implementing our solution for this client, we improved the existing PoC by properly collecting data and training new AI models. This allowed us to achieve an efficiency of over 96%. Since then, the company has been able to effectively count bacteria and scale their solution, avoiding the previous issues.
How important is data for the success of AI projects?
As I said, data is crucial for AI projects’ success. Without properly organized and labeled data, AI models cannot function properly. Data must be of high quality, meaning it must be accurate, complete, consistent, and up-to-date. Data management must also take into account ethical and regulatory aspects.
Can you give a specific example of a project where data quality affected the success of AI implementation?
I remember a case from a predictive maintenance project. The company stored machine cycle data in one system and component replacement data in another. Until we combine this data, we cannot build an effective predictive model. This example illustrates the challenge of integration, which is a very common problem blocking the acquisition of correct training data.
Another example is the previously mentioned project on recognizing and counting shrimp. Here, the challenge was to properly label and describe a large amount of data presented in thousands of photos. Our role was to accurately label the tank photos so that the model could recognize shrimp in various situations, even when they were partially covered. This shows how crucial it is to thoroughly understand the specifics of the business domain and properly prepare the data for training AI models.
Read also: How to Implement Predictive Maintenance Using Machine Learning?
Apart from technical roadblocks and lack of knowledge, what other challenges in AI projects can hinder AI implementation in companies?
The significant challenges in AI projects also include change management and legal regulations. Change management includes the adaptation of new technologies by employees and the integration of AI with business processes. Companies must cope with employees’ fear of losing their jobs and emphasize the benefits that AI brings, such as the automation of repetitive tasks and the elimination of errors.
Legal regulations can also limit the use of AI technology, especially in strictly regulated industries. For example, in the financial sector, AI-driven loan-granting algorithms must be transparent and clear to borrowers. This excludes the use of some more complex AI models. Although AI technology has the potential to precisely assess the client’s credit rating based on thousands of historical data, the mechanism will operate on the principle of the so-called black box – it will not provide full, transparent knowledge about the mechanics of rating analysis. In such a case, the use of artificial intelligence will not be possible.
There are also areas where AI technology can bring measurable benefits, but regulations do not allow for full automation of the process. The solution here is a partial implementation of AI. For example, in drug production, pharmaceutical companies can employ AI to detect contamination early on the production line, allowing production to be stopped quickly and losses to be minimized. However, final approval of samples must be done by a properly trained laboratory technician, under pharmaceutical law. This example illustrates that AI can still significantly reduce production costs, increase efficiency and maximize benefits, as long as companies apply AI in accordance with the law.
Read also: Industrial Application of AI In Microbiology
Companies have concerns about data security when using AI. Are these concerns justified?
Some of these concerns are certainly justified. Using tools like ChatGPT involves sending queries to external servers, which can lead to the disclosure of sensitive information. This is problematic for companies operating in industries with strict regulations regarding data security and confidentiality. However, some solutions limit this risk.
One solution is to use internal generative AI models installed on the company’s internal servers. We know of an example of a global pharmaceutical company that installed its own AI instance to complete documentation related to clinical trials, which ensured highly efficient work automation while maintaining the highest security standards.
We use this approach at NeuroSYS, installing AI models locally at customers’ premises so that data is not sent to the cloud. This is important when processing sensitive data. After analyzing their needs and limitations, companies can order an appropriate solution from us that will allow them to use artificial intelligence while ensuring AI’s compliance with regulations.
What AI trends and challenges do you see for scaling AI in companies?
The future of scaling AI in companies will be dominated by automation and the integration of AI with many business processes. Trends include the development of tools for automatic learning on larger amounts of data, improving interoperability between systems, and more advanced data management methods.
In the case of challenges in AI projects, it is primarily managing the complexity of AI systems, ensuring their scalability, and maintaining compliance with legal regulations. To effectively scale AI solutions and maximize their business value, companies must invest in IT infrastructure, team competencies, and appropriate data management strategies.
If you are facing challenges in AI projects or want to learn about the use cases and capabilities of AI-powered tools in your business, take advantage of the AI Discovery Workshop and start a project that will bring you the most optimal ROI:
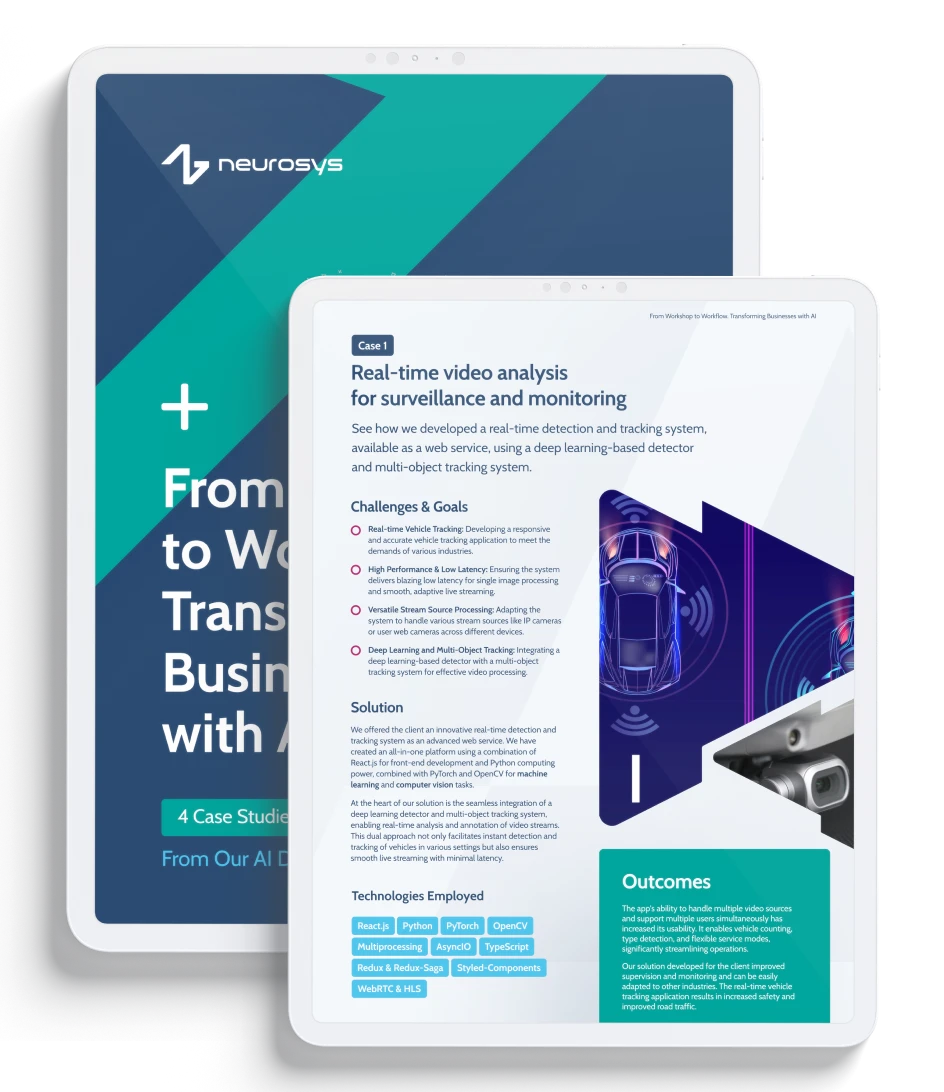